Elevator failure recognition method based on convolutional neural network
A convolutional neural network and fault identification technology, applied in the field of elevator fault identification based on convolutional neural network, can solve problems such as poor portability, no consideration of signal information, inability to adjust thresholds, etc., and achieve novel effects.
- Summary
- Abstract
- Description
- Claims
- Application Information
AI Technical Summary
Problems solved by technology
Method used
Image
Examples
Embodiment Construction
[0086] The specific implementation manners of the present invention will be further described in detail below in conjunction with the accompanying drawings.
[0087] Such as figure 1 As shown, the motion data of the elevator continuously collected by the sensor during normal operation or when a fault occurs is subjected to Kalman filtering (the fault data includes data when the elevator has different faults and different degrees of faults). Extract the z-direction acceleration and three-dimensional running attitude data in the elevator motion data, and divide the filtered signal at a rate of 4 seconds / segment. The four types of signals are transformed into time-spectrum diagrams by wavelet transform. Mark the fault type and fault degree for the samples in the sample set as the known labels of the data samples. Then the converted elevator motion images and their labels and other related information are stored in the original image database as the sample set of the convolution...
PUM
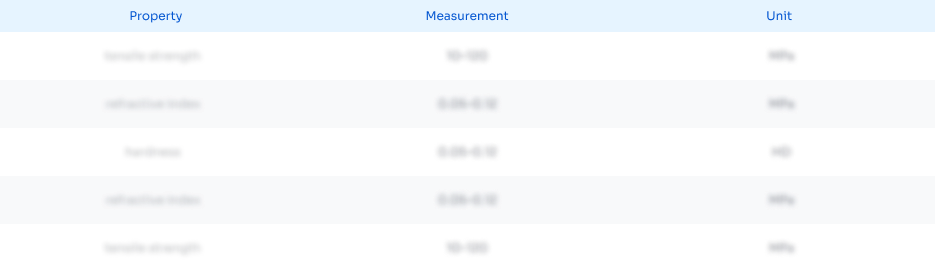
Abstract
Description
Claims
Application Information
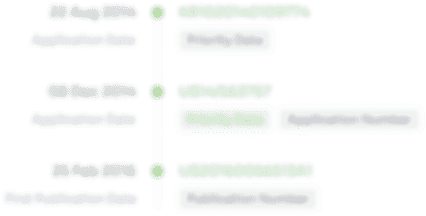
- R&D Engineer
- R&D Manager
- IP Professional
- Industry Leading Data Capabilities
- Powerful AI technology
- Patent DNA Extraction
Browse by: Latest US Patents, China's latest patents, Technical Efficacy Thesaurus, Application Domain, Technology Topic, Popular Technical Reports.
© 2024 PatSnap. All rights reserved.Legal|Privacy policy|Modern Slavery Act Transparency Statement|Sitemap|About US| Contact US: help@patsnap.com