Image Semantic Segmentation Method Based on Deep Fully Convolutional Network and Conditional Random Field
A Conditional Random Field, Fully Convolutional Network Technique
- Summary
- Abstract
- Description
- Claims
- Application Information
AI Technical Summary
Problems solved by technology
Method used
Image
Examples
Embodiment Construction
[0070] In order to make the technical means, creative features, goals and effects achieved by the present invention easy to understand, the present invention will be further described below in conjunction with specific illustrations and preferred embodiments.
[0071] Please refer to Figure 1 to Figure 3 As shown, the present invention provides a method for image semantic segmentation based on deep fully convolutional network and conditional random field, comprising the following steps:
[0072] S1. Construction of deep full convolutional semantic segmentation network model:
[0073] S11. The deep full convolution semantic segmentation network model includes a feature extraction module, a pyramid pooling module, and a pixel label prediction module. The feature extraction module extracts image parts by performing convolution, maximum pooling, and dilated convolution operations on the input image. feature; the pyramid pooling module performs different scale space pooling on th...
PUM
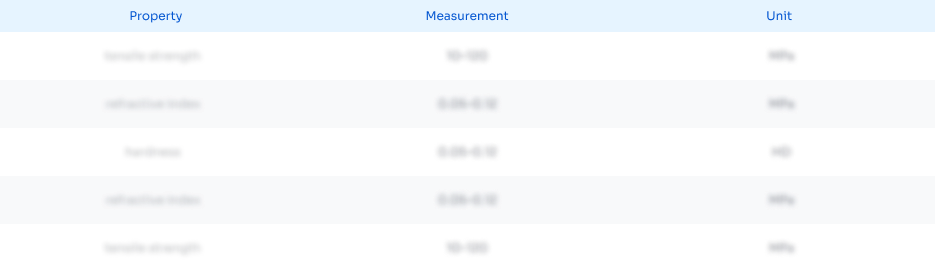
Abstract
Description
Claims
Application Information
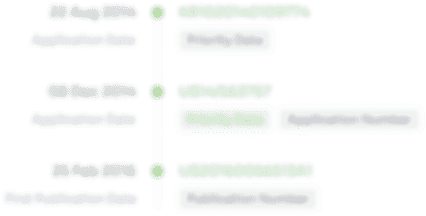
- R&D Engineer
- R&D Manager
- IP Professional
- Industry Leading Data Capabilities
- Powerful AI technology
- Patent DNA Extraction
Browse by: Latest US Patents, China's latest patents, Technical Efficacy Thesaurus, Application Domain, Technology Topic, Popular Technical Reports.
© 2024 PatSnap. All rights reserved.Legal|Privacy policy|Modern Slavery Act Transparency Statement|Sitemap|About US| Contact US: help@patsnap.com