Video fingerprint extraction method based on slowly-changing visual features
A video fingerprint and visual feature technology, applied in special data processing applications, instruments, electrical digital data processing, etc., can solve problems such as difficult to fully describe, complex information, etc., to avoid limitations, low computational complexity, and easy to implement Effect
- Summary
- Abstract
- Description
- Claims
- Application Information
AI Technical Summary
Problems solved by technology
Method used
Image
Examples
Embodiment 1
[0035] In order to realize a robust and efficient learning method for video fingerprints, an embodiment of the present invention proposes a method for extracting video fingerprints based on slowly changing visual features, see figure 1 and figure 2 , see the description below:
[0036] 101: Generate a random distorted image for each image in the training set, use the original image and the distorted image to train spatial features, and extract network parameters;
[0037] Among them, this step is specifically:
[0038] 1) Add random distortion to each image to obtain the corresponding distorted image;
[0039] The type of distortion may be set according to actual needs, and is not limited in this embodiment of the present invention.
[0040] 2) Normalize all images (that is, including the original image and the distorted image) to n×n, with a mean of 0 and a variance of 1, and different images as different classes, each image and its distorted version as the same class, by...
Embodiment 2
[0059] Taking an actual training process as an example, the scheme in Embodiment 1 is introduced in detail in combination with specific calculation formulas, and the video fingerprint learning method provided by the embodiment of the present invention is described in detail. See the following description for details:
[0060] 201: image preprocessing;
[0061] 15,000 images were randomly selected from ImageNet as the training data of the spatial feature extraction network, and the images were normalized to a standard size of 512×512, and filtered by mean value.
[0062] 202: Generate a random distorted version of the image;
[0063] Apply a random distortion or transformation to each image. The types of these distortions or transformations include: JPEG lossy compression, Gaussian noise, rotation, median filtering, histogram equalization, gamma correction, adding speckle noise, looping filtering. A total of 30,000 images.
[0064] 203: training spatial feature extraction ne...
Embodiment 3
[0079] Below in conjunction with specific experimental data, the scheme in embodiment 1 and 2 is carried out feasibility verification, see the following description for details:
[0080] In the above-mentioned embodiment 2, 600 video sequences downloaded from YouTube and another 201 video sequences from TRECVID total 801 videos are selected as test videos, and the videos are different in pairs and have no overlap with the training data. Nine common content-preserving distortions are applied to each video, and each distortion is selected to a different degree, as shown in the following table:
[0081] Table 1 Distortion types and parameter settings
[0082]
[0083] Each original video undergoes these distortions to generate 17 copy versions, and the total number of original videos and copies in the test library is 14418. Each video is normalized by the method of step 204 to obtain 32 × 32 × 20, which is divided into two video sequences of 32 × 32 × 10 and obtained by the m...
PUM
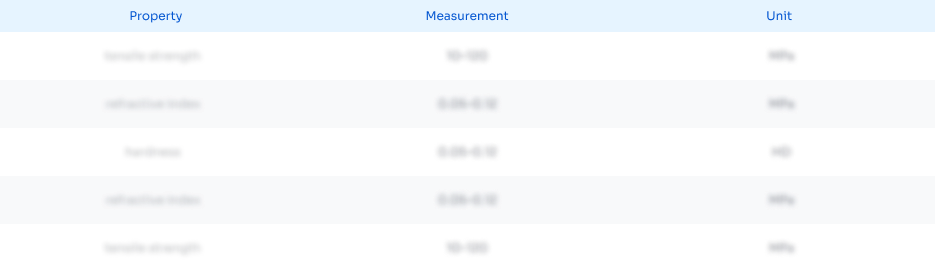
Abstract
Description
Claims
Application Information
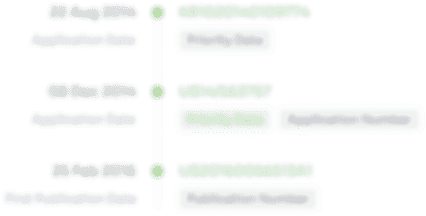
- R&D Engineer
- R&D Manager
- IP Professional
- Industry Leading Data Capabilities
- Powerful AI technology
- Patent DNA Extraction
Browse by: Latest US Patents, China's latest patents, Technical Efficacy Thesaurus, Application Domain, Technology Topic.
© 2024 PatSnap. All rights reserved.Legal|Privacy policy|Modern Slavery Act Transparency Statement|Sitemap