Automatic image annotation method based on cross-media sparse theme code
An automatic image annotation and cross-media technology, applied in the field of automatic image annotation based on cross-media sparse topic coding, can solve the problem that the probabilistic topic model cannot effectively control the sparsity of the underlying representation
- Summary
- Abstract
- Description
- Claims
- Application Information
AI Technical Summary
Problems solved by technology
Method used
Image
Examples
Embodiment 1
[0171] The specific implementation process of the present invention is divided into the following two stages:
[0172] 1. In the training model stage, 4,600 pictures crawled from the Wikipedia for schools course website are used as the training set, and 1-5 annotations are generated for each picture according to the text around the pictures on the original webpage. Such as figure 2 , using this dataset for model training includes the following steps:
[0173] Step 101: Split the 4600 training images into some 20×20 tiles by sliding a 20-pixel wide window, and extract the 128-dimensional SIFT (Scale Invariant FeatureTransform) description from these 20×20 grayscale tiles symbol, and add an additional 36-dimensional color descriptor to the SIFT descriptor, so each picture is represented as an n×164-dimensional matrix (n is the number of SIFT feature points), or 164-dimensional features Collection of vectors. Among them, Scale-invariant feature transform (Scale-invariant feat...
PUM
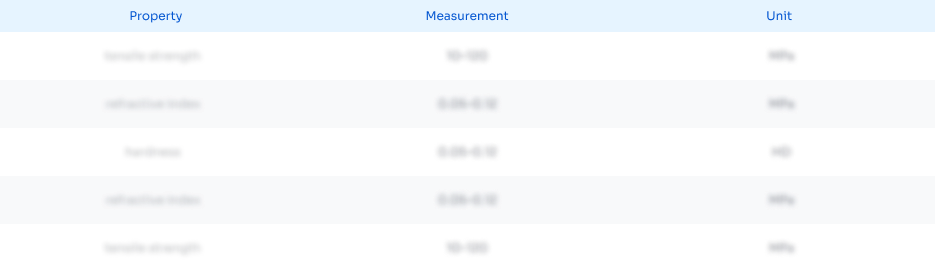
Abstract
Description
Claims
Application Information
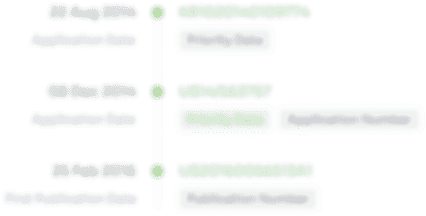
- R&D Engineer
- R&D Manager
- IP Professional
- Industry Leading Data Capabilities
- Powerful AI technology
- Patent DNA Extraction
Browse by: Latest US Patents, China's latest patents, Technical Efficacy Thesaurus, Application Domain, Technology Topic, Popular Technical Reports.
© 2024 PatSnap. All rights reserved.Legal|Privacy policy|Modern Slavery Act Transparency Statement|Sitemap|About US| Contact US: help@patsnap.com