Multi-objective optimization improved genetic algorithm based on dynamic weight M-TOPSIS multi-attribute decision-making
An improved genetic algorithm and multi-objective optimization technology, applied in the field of optimization design, to achieve the effect of multiple selection opportunities, good engineering applicability, and expanded search range
- Summary
- Abstract
- Description
- Claims
- Application Information
AI Technical Summary
Problems solved by technology
Method used
Image
Examples
Embodiment 1
[0072] Aiming at the internal ballistic process of artillery firing, the optimization algorithm of the present invention is used to optimize the launch charge parameters and internal bore structure parameters to obtain a better internal ballistic design scheme. The optimized design variables include: propellant mass ω i , gunpowder thickness e i , gunpowder aperture d 0i , gunpowder length l ci , The chamber volume V of the inner bore structure 0 , constituting the design variable vector X, where the subscript i=1,2, i=1 means thin gunpowder, i=2 means thick gunpowder. The objective function is the muzzle pressure P at the end of the internal trajectory g , charge utilization coefficient η ω , working volume utilization factor η g . The constraint function is projectile velocity V g , the maximum pressure P m , the relative position η of the end of propellant combustion k .
[0073] According to the main design requirements of the inner ballistic, the mathematical m...
PUM
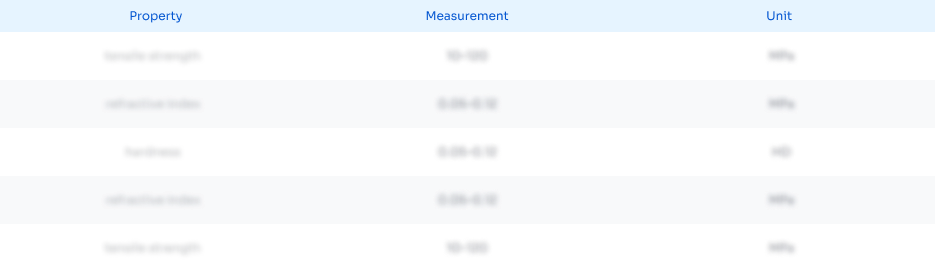
Abstract
Description
Claims
Application Information
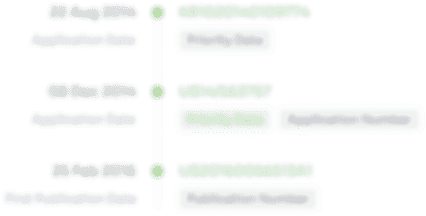
- R&D Engineer
- R&D Manager
- IP Professional
- Industry Leading Data Capabilities
- Powerful AI technology
- Patent DNA Extraction
Browse by: Latest US Patents, China's latest patents, Technical Efficacy Thesaurus, Application Domain, Technology Topic, Popular Technical Reports.
© 2024 PatSnap. All rights reserved.Legal|Privacy policy|Modern Slavery Act Transparency Statement|Sitemap|About US| Contact US: help@patsnap.com