Rolling Bearing Fault Diagnosis Method Based on Bayesian Residual Transformation-Singular Value Decomposition-Gaussian Mixture Hidden Markov Model Framework
A singular value decomposition and Gaussian mixture technology, applied in the field of mechanical equipment fault diagnosis, which can solve problems such as false signals, data sequence influence, and effective value reduction.
- Summary
- Abstract
- Description
- Claims
- Application Information
AI Technical Summary
Problems solved by technology
Method used
Image
Examples
specific Embodiment
[0071] The combined framework of Bayesian residual transformation-singular value decomposition-Gaussian mixed Markov model is used to process four different types of fault signals. The four types of faults include inner ring faults, outer ring faults, rolling element faults and cage faults. The first step: use Bayesian residual transformation to decompose the fault signal, obtain residual signals on different scales, perform threshold processing and denoising on high-scale residual signals, and reconstruct the signal; second step: singularize the reconstructed signal value decomposition to improve the stability of fault features, and use the obtained singular value vectors as fault features; the third step: divide the pre-processed 4 different fault signals into 480 samples, and use the Gaussian mixture hidden Markov model to analyze the 480 samples for training and classification. classification result Figure 4 As shown, through calculation, the recognition rate of the firs...
comparative example 1
[0083] Rolling Bearing Fault Diagnosis Method Based on Bayesian Residual Transform and Gaussian Mixture Hidden Markov Model. In this comparative case, the singular value decomposition of the second step of the rolling bearing fault diagnosis method in the present invention is removed. First, the Bayesian residual transform is used to decompose the fault signal to obtain residual signals on different scales, and the residual signals on high scales are thresholded, de-noised, and the signal is reconstructed; secondly, the four different The fault signal is divided into 480 samples, and the Gaussian mixture hidden Markov model is used to train and classify these 480 samples. classification result Figure 4 As shown, through calculation, the recognition rate of the first type of fault is 87.5%, the recognition rate of the second type of fault is 83.3%, the recognition rate of the third type of fault is 90%, and the recognition rate of the fourth type of fault is 80%.
[0084] It...
comparative example 2
[0095] Fault Diagnosis Method for Rolling Bearings Based on Singular Value Decomposition and Gaussian Mixture Hidden Markov Model. In this comparison case, the Bayesian residual transformation in the first step of the rolling bearing fault diagnosis method of the present invention is removed. First, the original bearing fault signal is subjected to singular value decomposition using the singular value decomposition method to extract the singular value eigenvectors; secondly, the extracted singular value eigenvectors of 4 different faults are divided into 480 samples, and the Gaussian mixture hidden Markov model is used These 480 samples are trained and classified. classification result Figure 4 As shown, through calculation, the recognition rate of the first type of fault is 66.7%, the recognition rate of the second type of fault is 54.2%, the recognition rate of the third type of fault is 70%, and the recognition rate of the fourth type of fault is 52.5%.
[0096] It can b...
PUM
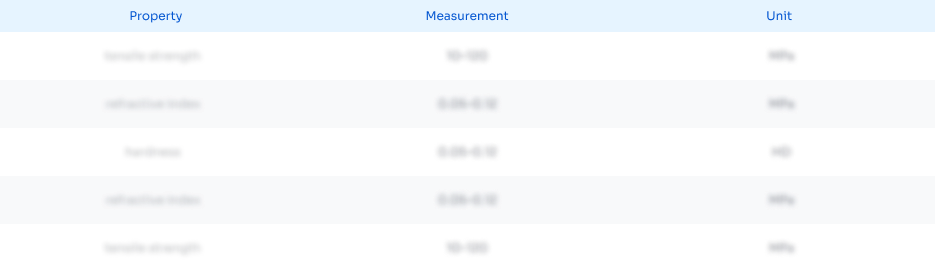
Abstract
Description
Claims
Application Information
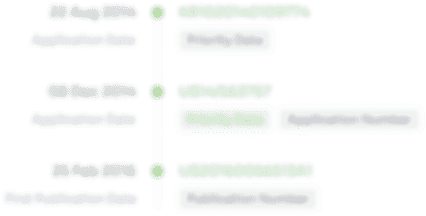
- R&D Engineer
- R&D Manager
- IP Professional
- Industry Leading Data Capabilities
- Powerful AI technology
- Patent DNA Extraction
Browse by: Latest US Patents, China's latest patents, Technical Efficacy Thesaurus, Application Domain, Technology Topic, Popular Technical Reports.
© 2024 PatSnap. All rights reserved.Legal|Privacy policy|Modern Slavery Act Transparency Statement|Sitemap|About US| Contact US: help@patsnap.com