Fault diagnosis method for planetary gear box based on data-driven quantitative characteristic multi-granularities
A planetary gearbox, data-driven technology, applied in the direction of instruments, character and pattern recognition, computer components, etc., can solve the problem of incomplete fault diagnosis information of planetary gearboxes, achieve clear pattern recognition strategies, reduce required data, Effect of Accurate Fault Diagnosis Results
- Summary
- Abstract
- Description
- Claims
- Application Information
AI Technical Summary
Problems solved by technology
Method used
Image
Examples
specific Embodiment approach 1
[0030] Specific implementation mode one: combine figure 1 Describe this embodiment, the multi-granularity planetary gearbox fault diagnosis method based on data-driven quantitative features, is characterized in that the method includes the following steps:
[0031] Step 1. According to the collected characteristic signals of typical faulty planetary gearboxes, extract fault diagnosis features and establish an incomplete fault diagnosis information system;
[0032] Step 2: Analyze the incomplete fault diagnosis information system by using the data-driven quantitative feature relationship, calculate the feature similarity between all instances, and obtain a feature set that satisfies the data-driven quantitative feature relationship;
[0033] Step 3. Using the attribute reduction algorithm based on the pessimistic data-driven quantitative feature multi-granularity model to extract fault diagnosis decision rules;
[0034] Step 4: Construct a naive Bayesian classifier model accor...
specific Embodiment approach 2
[0035] Embodiment 2: The difference between this embodiment and Embodiment 1 is that the feature similarity between instances in the step 2 is defined as follows:
[0036] For an incomplete information system S=(U,A=C∪D,V,f), U is the instance set, A is the attribute set, C is the symptom attribute set, D is the decision attribute set, V is the value range of A, f is a mapping function, let the attribute set right in Indicates all the different known attribute values of the instance on the attribute b, Indicates that the attribute value of the instance on the attribute b is b i the number of instances of , then Feature similarity VR on attribute set B B (x,y) calculation formula is:
[0037] VR B (x,y)=Π b∈B R b (x,y)·N B (x,y) (1)
[0038] Among them, N B (x, y) represents the proportion of missing attribute values in instance x and y, R b (x, y) represents the feature similarity between instances x and y on attribute b, |X| represents the cardinality ...
specific Embodiment approach 3
[0044]Embodiment 3: The difference between this embodiment and Embodiment 1 or 2 is that the definition of data-driven quantitative feature relationship in Step 2 is as follows:
[0045] For an incomplete information system S=(U,A=C∪D,V,f), U is the instance set, A is the attribute set, C is the symptom attribute set, D is the decision attribute set, V is the value range of A, f is a mapping function, let the attribute set Then the data-driven quantitative feature relationship is:
[0046] VR(B)={(x,y)∈U×U|y∈K B (x), VR B (x,y)≥α} (4)
[0047] Among them, α is the threshold. If the threshold α is too large, the feature set that satisfies the data-driven quantitative feature relationship contains too few instances; if the threshold α is too small, the feature set that satisfies the data-driven quantitative feature relationship contains too many instances. In order to avoid the occurrence of the above situation, the average value of the minimum feature similarity between a...
PUM
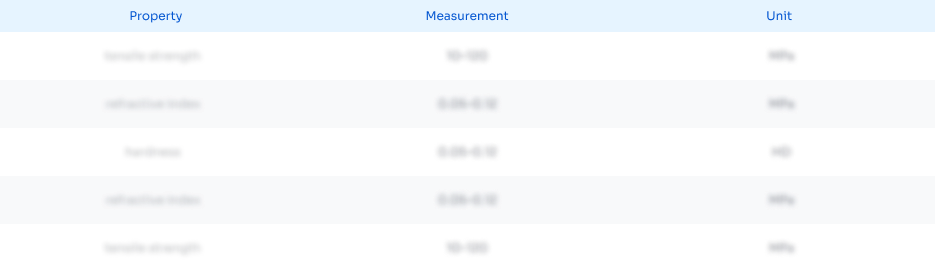
Abstract
Description
Claims
Application Information
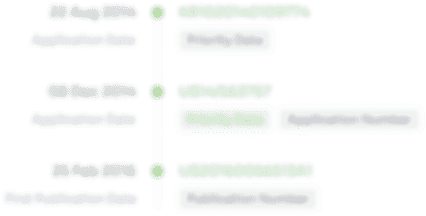
- Generate Ideas
- Intellectual Property
- Life Sciences
- Materials
- Tech Scout
- Unparalleled Data Quality
- Higher Quality Content
- 60% Fewer Hallucinations
Browse by: Latest US Patents, China's latest patents, Technical Efficacy Thesaurus, Application Domain, Technology Topic, Popular Technical Reports.
© 2025 PatSnap. All rights reserved.Legal|Privacy policy|Modern Slavery Act Transparency Statement|Sitemap|About US| Contact US: help@patsnap.com