Convolutional neural network relation classification method combining forward and reverse instances
A convolutional neural network and relationship classification technology, applied in the field of relationship extraction and classification, can solve problems such as good classification effect, high training efficiency, and difficulty in obtaining classification effect, so as to achieve good classification effect, strong robustness, and improve the final classification effect of effect
- Summary
- Abstract
- Description
- Claims
- Application Information
AI Technical Summary
Problems solved by technology
Method used
Image
Examples
Embodiment Construction
[0050] The present invention relates to a relation extraction technology in information extraction, in particular to a relation classification method based on machine learning. The mainstream method of the existing relation extraction technology is realized by the relation classification method. The present invention utilizes the existing word vector training technology and grammatical analysis tool to represent the text, and on this basis, we carry out the relationship classification based on the neural network. The invention mainly includes a neural network feature extraction and representation module, and a combination classification module of multiple representations. The present invention mainly realizes the relation extraction technology through the relation classification method. Relation extraction, that is, identifying and generating semantic relations between entities from unformatted text. For example, enter the text "Financial stress is one of the main causes of d...
PUM
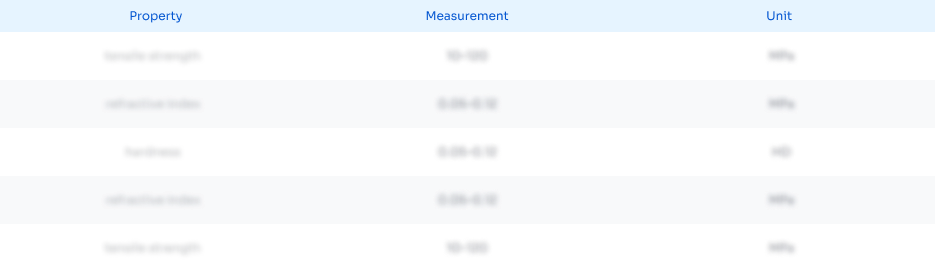
Abstract
Description
Claims
Application Information
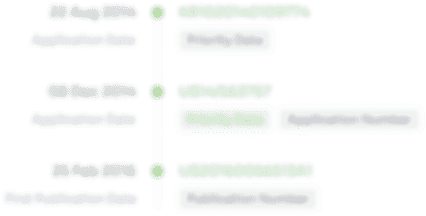
- R&D Engineer
- R&D Manager
- IP Professional
- Industry Leading Data Capabilities
- Powerful AI technology
- Patent DNA Extraction
Browse by: Latest US Patents, China's latest patents, Technical Efficacy Thesaurus, Application Domain, Technology Topic, Popular Technical Reports.
© 2024 PatSnap. All rights reserved.Legal|Privacy policy|Modern Slavery Act Transparency Statement|Sitemap|About US| Contact US: help@patsnap.com