Dictionary learning static image lossy compression method based on minimum quantization error criterion
A technology of quantization error and dictionary learning, which is applied in image coding, image data processing, character and pattern recognition, etc., can solve the problem of large quantization error, achieve the effect of small quantization error and reduce coding cost
- Summary
- Abstract
- Description
- Claims
- Application Information
AI Technical Summary
Problems solved by technology
Method used
Image
Examples
Embodiment Construction
[0019] The specific steps of the dictionary learning static image lossy compression method based on the minimum quantization error criterion of the present invention are as follows:
[0020] 1. Image segmentation and normalization.
[0021] For all training images, they are first divided into 16×16 image blocks according to the raster scanning order, and the translation amount is 2 Then for each image block b i Carry out normalization with reference to formula (1)
[0022]
[0023] Finally, straighten the image patch into a vector Constitutes the input signal for dictionary learning.
[0024] 2. Image block clustering.
[0025] In order to ensure the purity of the learned dictionary, it is necessary to cluster the image blocks first, so that each class has similar image blocks. Since the training image is overlapped and divided into blocks, the number of image blocks obtained is very large, so firstly, 10% of the image blocks are randomly selected from all image bloc...
PUM
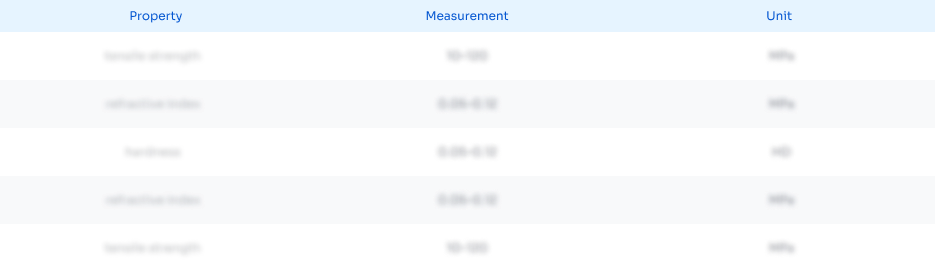
Abstract
Description
Claims
Application Information
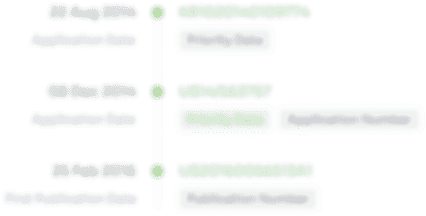
- Generate Ideas
- Intellectual Property
- Life Sciences
- Materials
- Tech Scout
- Unparalleled Data Quality
- Higher Quality Content
- 60% Fewer Hallucinations
Browse by: Latest US Patents, China's latest patents, Technical Efficacy Thesaurus, Application Domain, Technology Topic, Popular Technical Reports.
© 2025 PatSnap. All rights reserved.Legal|Privacy policy|Modern Slavery Act Transparency Statement|Sitemap|About US| Contact US: help@patsnap.com