Dynamic neural network model training method and device
A technology of dynamic neural network and neural network model, which is applied in the training field of dynamic neural network model, can solve problems such as the inability to describe the relationship between systems, and achieve the effects of reducing training difficulty, improving algorithm efficiency, and reducing design difficulty
- Summary
- Abstract
- Description
- Claims
- Application Information
AI Technical Summary
Problems solved by technology
Method used
Image
Examples
Embodiment 2
[0058] Based on the method in Embodiment 1, the present invention also provides a dynamic neural network model training device, which includes a model building module, a model evaluation module and a model optimization module. in:
[0059] The model building module is used for the initialization of the neural network model. The original one-dimensional data is input to the first layer of neurons, and the corresponding output value is the feature of this layer; the number of neuron layers is increased, and the features output by the upper layer are used as the next layer of neurons Element input to get the characteristics of the corresponding layer, repeat this step until the number of layers reaches the preset value;
[0060] In the neural network model, neurons in the same layer have the same dynamic structure, and the dynamic structures of neurons in different layers can be the same or different; there is no connection between neurons in the same layer, and the connection mo...
Embodiment 3
[0073] Taking the speech data as an example, the speech data is a time series data, and the initial data is a very long time-related vector, and the speech data is used as the training data to train the neural network model.
[0074] Input the voice data into the initialized neural network model, shorten the length of the vector to a certain value (for example, the length k to 50) layer by layer, and then use BP backpropagation to determine the full range of the layer and the number of discussion topics. Connect the weight coefficients; test the performance on the verification set, then add a layer of neurons to obtain new features, use the BP backpropagation algorithm to train the corresponding weight parameters, and test whether the performance has improved significantly on the verification set, and if so, then Continue to increase the neural network layer, otherwise, stop the increase in the number of model layers. If the number of layers increases to a certain threshold an...
PUM
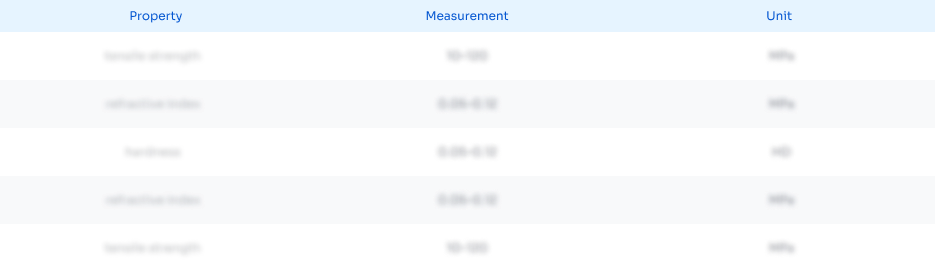
Abstract
Description
Claims
Application Information
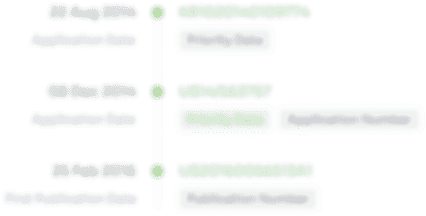
- R&D Engineer
- R&D Manager
- IP Professional
- Industry Leading Data Capabilities
- Powerful AI technology
- Patent DNA Extraction
Browse by: Latest US Patents, China's latest patents, Technical Efficacy Thesaurus, Application Domain, Technology Topic, Popular Technical Reports.
© 2024 PatSnap. All rights reserved.Legal|Privacy policy|Modern Slavery Act Transparency Statement|Sitemap|About US| Contact US: help@patsnap.com