Image super-resolution reconstruction method based on learning
A technology of super-resolution reconstruction and image reconstruction, applied in the field of image and video, can solve the problems of inability to realize real-time super-resolution reconstruction, low reconstruction efficiency, etc., to reduce the number of dictionary atoms, low cost, and improve computing. The effect of efficiency
- Summary
- Abstract
- Description
- Claims
- Application Information
AI Technical Summary
Problems solved by technology
Method used
Image
Examples
Embodiment 1
[0045] See figure 1 , figure 1 This is a schematic diagram of a learning-based image super-resolution reconstruction method provided by an embodiment of the present invention. The method includes the following steps:
[0046] Step 1. According to the degraded model blur and N times downsampling, process the first resolution image to form the second resolution image;
[0047] Step 2. Train the first resolution image and the second resolution image to form an image learning dictionary;
[0048] Step 3. Perform block division on the image to be reconstructed to form multiple image blocks to be reconstructed;
[0049] Step 4. Using an edge extraction algorithm, determine a fixed threshold of image information according to the second resolution image;
[0050] Step 5. If the information amount of the image block to be reconstructed is greater than the fixed threshold, perform image reconstruction on the image block to be reconstructed according to the image learning dictionary to form a fir...
Embodiment 2
[0080] This embodiment describes the technical solution of the present invention in detail on the basis of the foregoing embodiment. Specifically, the method includes:
[0081] Step 1: Using a large number of samples of high-resolution images (ie, the first resolution image), the high-resolution images are subjected to blur processing and N-fold down-sampling according to the modified degradation model to obtain the corresponding low-resolution images (ie, the first resolution image). Two-resolution image) sample.
[0082] Step 2: For the low-resolution image obtained in step 1, the image features are extracted through the feature extraction algorithm to obtain the high-resolution feature information X of the space target s (I.e. first resolution feature information) and low resolution feature information Y s (Ie the second resolution feature information).
[0083] Step 3: Use the K-SVD algorithm to jointly train the feature information to obtain a high-resolution image learning dic...
Embodiment approach
[0101] S1: Perform block segmentation on a large number of low-resolution image samples to obtain image blocks;
[0102] S2: Use an edge extraction algorithm to extract edge information of low-resolution image blocks, and count the information volume of each low-resolution image block and the distribution of information volume of all low-resolution image blocks;
[0103] S3: Select the value with the highest amount of information in the low-resolution image block, obtain the pixel value of the low-resolution image block as F1, f=F1 / 4, then f*40% <= threshold <=f*60%, take several representative thresholds in this range, for example, you can take the following representative thresholds: f*40%, f*45%, f*50%, f*55%, f*60%, calculate each The learning-based sparse representation of each threshold point corresponds to the reconstruction time of the image super-resolution reconstruction algorithm and the resolution of the reconstructed image, which can be judged according to subjective ev...
PUM
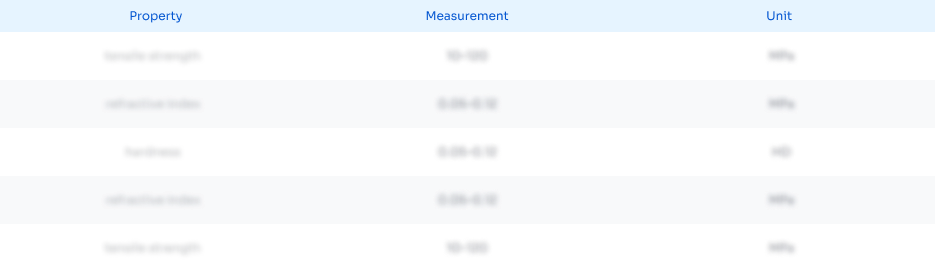
Abstract
Description
Claims
Application Information
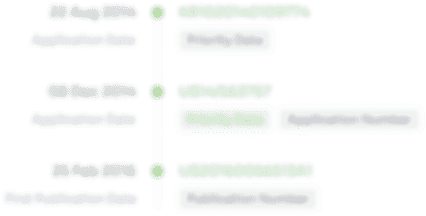
- R&D Engineer
- R&D Manager
- IP Professional
- Industry Leading Data Capabilities
- Powerful AI technology
- Patent DNA Extraction
Browse by: Latest US Patents, China's latest patents, Technical Efficacy Thesaurus, Application Domain, Technology Topic, Popular Technical Reports.
© 2024 PatSnap. All rights reserved.Legal|Privacy policy|Modern Slavery Act Transparency Statement|Sitemap|About US| Contact US: help@patsnap.com