Deep convolutional neural network-based stockyard smoke monitoring and online model updating method
A neural network model and deep convolution technology, applied in the field of smoke monitoring, can solve the problems of not being able to better describe the nature of smoke, poor adaptability to environmental changes, and difficulty in eliminating interference from smoke-like objects, so as to reduce property losses and improve High detection accuracy and high stability
- Summary
- Abstract
- Description
- Claims
- Application Information
AI Technical Summary
Problems solved by technology
Method used
Image
Examples
Embodiment Construction
[0028] The present invention will be further described below in conjunction with specific drawings.
[0029] Such as figure 1 As shown, the stackyard smoke monitoring and online model update method based on the deep convolutional neural network of the present invention comprises the following steps:
[0030] (1) the smog video that collects is converted into picture sequence, does not have special requirement to conversion tool and picture format, and in the present embodiment, conversion tool adopts self-made python tool, and picture format is jpg format;
[0031] (2) Mark the area where there is smoke in the picture in the form of a rectangular frame, and mark it with the class of Smoke (such as figure 2 As shown), for the smoke-like object, the class label is likesmoke, and there is no special requirement for the labeling tool. In this embodiment, the labeling tool adopts a self-made python tool;
[0032] (3) The position of the rectangular frame in the marked picture an...
PUM
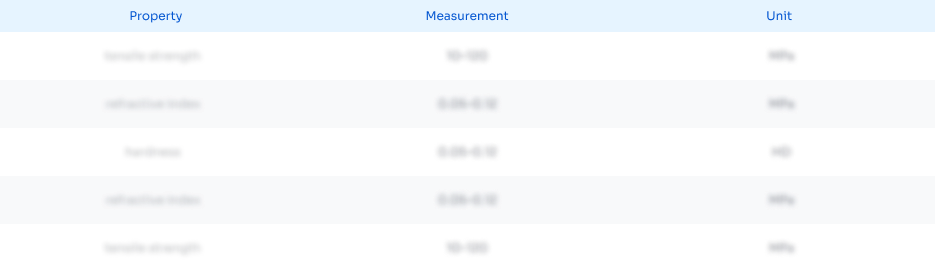
Abstract
Description
Claims
Application Information
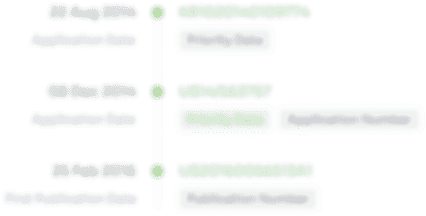
- R&D Engineer
- R&D Manager
- IP Professional
- Industry Leading Data Capabilities
- Powerful AI technology
- Patent DNA Extraction
Browse by: Latest US Patents, China's latest patents, Technical Efficacy Thesaurus, Application Domain, Technology Topic, Popular Technical Reports.
© 2024 PatSnap. All rights reserved.Legal|Privacy policy|Modern Slavery Act Transparency Statement|Sitemap|About US| Contact US: help@patsnap.com