Data analysis and combination primary function nerve network-based wind power prediction method
A technology of wind power forecasting and neural network, applied in AC network circuits, forecasting, data processing applications, etc., can solve problems such as long forecasting time, low forecasting accuracy, and short forecasting lead time
- Summary
- Abstract
- Description
- Claims
- Application Information
AI Technical Summary
Problems solved by technology
Method used
Image
Examples
Embodiment Construction
[0074] The wind power prediction method of the present invention will be further described below in combination with specific examples.
[0075] (1) The 2014 annual output power sample data of a 1.5MW wind farm in Xinjiang is selected, and the time resolution is selected to be 10 minutes. The data on the 5th and 15th of each month are used as training samples, and the data on the 25th are used as forecast data. 5184 data as training and prediction data points, such as figure 2 .
[0076] (2) Parameter setting: Compared with the traditional neural network prediction model, each sub-network of the combined basis function neural network of the present invention avoids the selection of the network structure, and the input of the model depends entirely on the result of the phase space reconstruction; the state The parameters of the transfer algorithm are set as follows: the execution search group size (SE) is set to 80, the number of iterations is 500, the intermittent communicat...
PUM
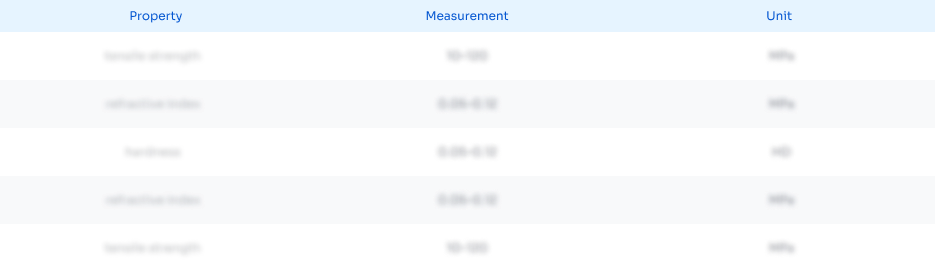
Abstract
Description
Claims
Application Information
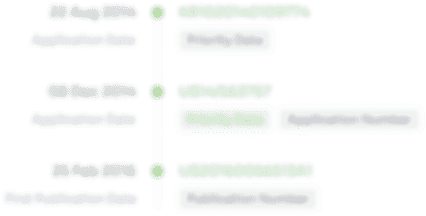
- R&D Engineer
- R&D Manager
- IP Professional
- Industry Leading Data Capabilities
- Powerful AI technology
- Patent DNA Extraction
Browse by: Latest US Patents, China's latest patents, Technical Efficacy Thesaurus, Application Domain, Technology Topic, Popular Technical Reports.
© 2024 PatSnap. All rights reserved.Legal|Privacy policy|Modern Slavery Act Transparency Statement|Sitemap|About US| Contact US: help@patsnap.com