Weight clustering and under-sampling-based unbalanced data classification method
A data classification and under-sampling technology, applied in the computer field, can solve the problems of loss of majority sample information and uncertain compensation degree, and achieve the effects of improving classification accuracy, optimizing random under-sampling, and increasing computing overhead
- Summary
- Abstract
- Description
- Claims
- Application Information
AI Technical Summary
Problems solved by technology
Method used
Image
Examples
Embodiment 1
[0078] In this implementation, the data used is 1000 pieces of two-dimensional data generated manually, and the ratio of the majority class to the minority class is 9:1. in figure 2 (a) is the general unbalanced data with a clear boundary between the majority class and the minority class. figure 2 (b) is the data where the majority class overlaps with the minority class. figure 2 (c) Imbalanced data showing separation of minority class subsets. Among them, 'x' indicates that the point is a majority class sample, and 'o' indicates that the point is a minority class sample. Table 1 shows the algorithm used in the experimental comparison. In this implementation, under the unbalanced data situation of these three distributions, the clustering method based on the sample weight variance proposed by the present invention is compared with K-Means (CEU) and the hierarchical clustering method. Clustering (EHCU) effect comparison experiment, the sample points of the same gray level...
Embodiment 2
[0084] In this implementation, 22 groups of KEEL data with different practical application backgrounds are selected as experimental test data. In the selected data set, the smallest ratio of the number of majority and minority classes is 9.09, and the largest is 128. For data with multiple categories, combine some categories or take only two categories. The experimental results are shown in Table 2. In order to make the results more reliable, the experiment conducts 5 experiments for each validation of each dataset and takes the average of the AUC results. Table 2 shows the experimental results of each comparison algorithm and the algorithm proposed in this paper on 22 imbalanced datasets.
[0085] Table 2 AUC index experimental results
[0086]
[0087] The results show that the algorithm proposed by the present invention has better performance than other algorithms on more data sets, and the comprehensive average AUC value is the largest. Algorithms improved by an ave...
PUM
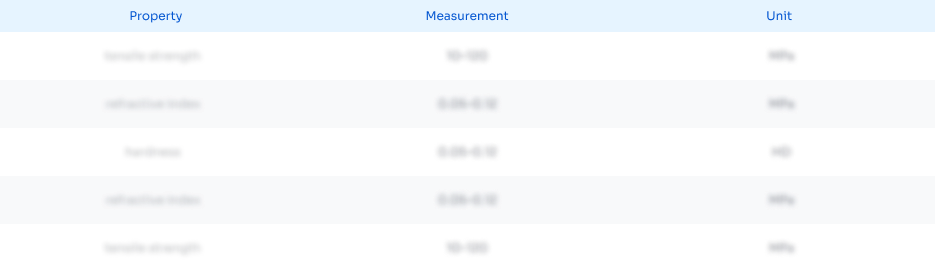
Abstract
Description
Claims
Application Information
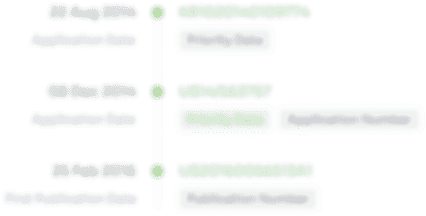
- R&D Engineer
- R&D Manager
- IP Professional
- Industry Leading Data Capabilities
- Powerful AI technology
- Patent DNA Extraction
Browse by: Latest US Patents, China's latest patents, Technical Efficacy Thesaurus, Application Domain, Technology Topic, Popular Technical Reports.
© 2024 PatSnap. All rights reserved.Legal|Privacy policy|Modern Slavery Act Transparency Statement|Sitemap|About US| Contact US: help@patsnap.com