Sparse Gaussian process regression method for visual mapping
A Gaussian process regression, vision technology, applied in the field of vision mapping, can solve the problem of no feature selection function, high-dimensional overfitting, etc.
- Summary
- Abstract
- Description
- Claims
- Application Information
AI Technical Summary
Problems solved by technology
Method used
Image
Examples
Embodiment Construction
[0069] Implementation language: Matlab, C / C++
[0070] Hardware platform: Intel core2E7400+4G DDR RAM
[0071] Software platform: Matlab2012a, VisualStdio2010
[0072] According to the method of the present invention, first collect a certain number of images and record the corresponding target values of these images, according to the patent of the present invention, utilize Matlab or C++ language to write the sparse Gaussian process regression model program, and train the corresponding model of the present invention on the collected data parameters; then install the camera in various application scenarios to collect the original image, and extract the gradient orientation histogram features; according to the previously trained parameters, the corresponding output target value of the image can be estimated. The method of the present invention can be used for visual mapping problems in various scenarios.
[0073] A sparse Gaussian process regression method for visual mapping...
PUM
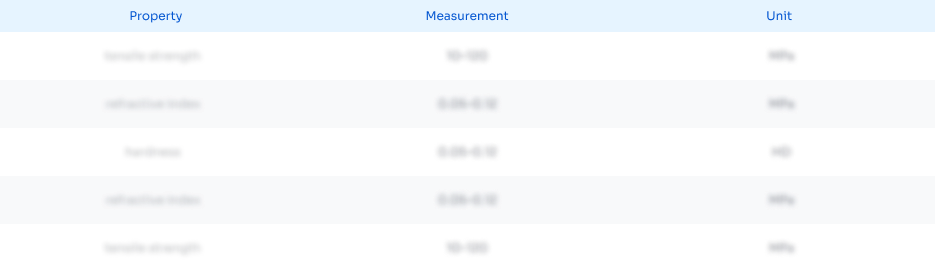
Abstract
Description
Claims
Application Information
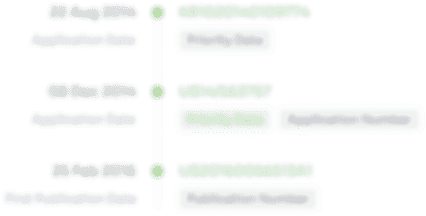
- Generate Ideas
- Intellectual Property
- Life Sciences
- Materials
- Tech Scout
- Unparalleled Data Quality
- Higher Quality Content
- 60% Fewer Hallucinations
Browse by: Latest US Patents, China's latest patents, Technical Efficacy Thesaurus, Application Domain, Technology Topic, Popular Technical Reports.
© 2025 PatSnap. All rights reserved.Legal|Privacy policy|Modern Slavery Act Transparency Statement|Sitemap|About US| Contact US: help@patsnap.com