Traffic flow sequence classification method based on density peak value clustering
A technology of peak density and traffic flow, applied in the field of traffic control research, it can solve the problems such as similarity in flow change laws, large computational time complexity, and inability to automatically optimize the number of clusters.
- Summary
- Abstract
- Description
- Claims
- Application Information
AI Technical Summary
Problems solved by technology
Method used
Image
Examples
Embodiment Construction
[0056] Taking the 24-day traffic sequence of an intersection in a city as an example, classify the 24-day data. For the specific implementation process, see figure 1 .
[0057] 1. Divide the total flow sequence into 24 subsequences in units of days, and calculate the local density of each subsequence:
[0058] (1) In the 24 subsequences, calculate the similarity between every two subsequences, record 24 subsequences as X=x 1 ,x 2 ,...x n ;
[0059] (2) For any subsequence i, the subsequence is divided into several segments at intervals of fixed periods, and the segment sequence is x i =x i (1), x i (2),...,x i (N); usually the fixed period is 5 minutes, 10 minutes or 15 minutes.
[0060] ①Calculate the corresponding Euclidean distance d between subsequences i and j ij :
[0061]
[0062] ②Calculate the average of all subsequence distances:
[0063]
[0064] ③ Calculate the variance of the Euclidean distance between subsequence i and other subsequences:
[006...
PUM
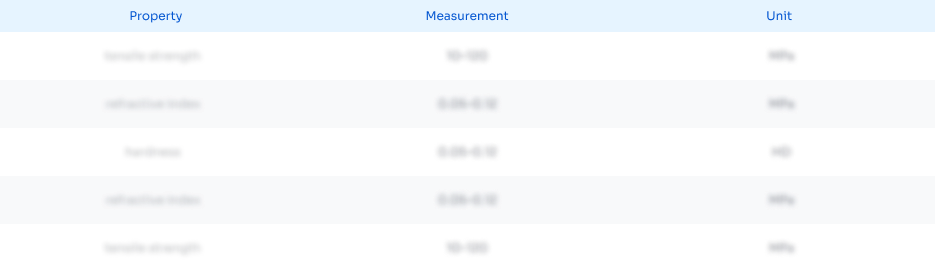
Abstract
Description
Claims
Application Information
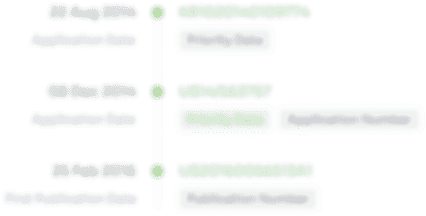
- R&D Engineer
- R&D Manager
- IP Professional
- Industry Leading Data Capabilities
- Powerful AI technology
- Patent DNA Extraction
Browse by: Latest US Patents, China's latest patents, Technical Efficacy Thesaurus, Application Domain, Technology Topic, Popular Technical Reports.
© 2024 PatSnap. All rights reserved.Legal|Privacy policy|Modern Slavery Act Transparency Statement|Sitemap|About US| Contact US: help@patsnap.com