Improved self-adaptive multi-dictionary learning image super-resolution reconstruction method
A technology of super-resolution reconstruction and dictionary learning, which is applied in the field of image processing, can solve the problems of low computation, reduced reconstruction effect, checkerboard and ringing effects, etc., and achieve good image effect, peak signal-to-noise ratio and structural self-similarity The effect of improving quantitative indicators
- Summary
- Abstract
- Description
- Claims
- Application Information
AI Technical Summary
Problems solved by technology
Method used
Image
Examples
Embodiment Construction
[0051] The present invention will be further described in detail below in combination with specific embodiments.
[0052] The present invention realizes the image super-resolution reconstruction method based on adaptive multi-dictionary learning and structural self-similarity through the following steps, and the specific steps are as follows:
[0053] Step 1: Determine the downsampling matrix D and the fuzzy matrix B according to the image degradation process;
[0054] Step 2: Use image self-similarity to build a pyramid, use the upper image of the pyramid and natural images as samples for dictionary learning, and use the PCA method to build various dictionaries and take the top image of the pyramid as the initial reconstructed image In order to get the missing high-frequency information, it is necessary to obtain a dictionary containing high-frequency information. The samples used for dictionary training in the present invention are the image to be processed itself and th...
PUM
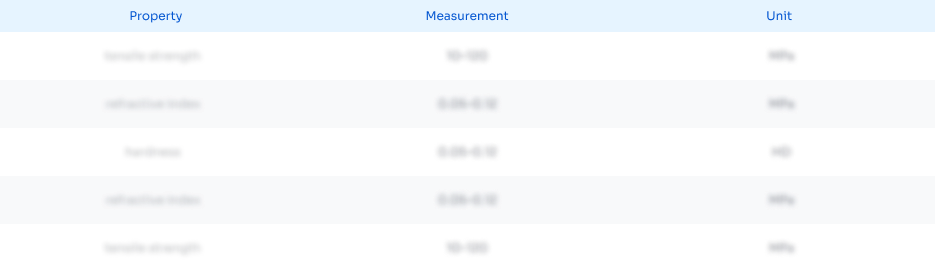
Abstract
Description
Claims
Application Information
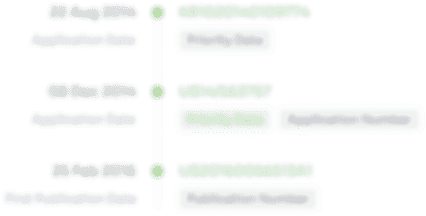
- R&D Engineer
- R&D Manager
- IP Professional
- Industry Leading Data Capabilities
- Powerful AI technology
- Patent DNA Extraction
Browse by: Latest US Patents, China's latest patents, Technical Efficacy Thesaurus, Application Domain, Technology Topic, Popular Technical Reports.
© 2024 PatSnap. All rights reserved.Legal|Privacy policy|Modern Slavery Act Transparency Statement|Sitemap|About US| Contact US: help@patsnap.com