Vehicle classifier training method
A training method and classifier technology, applied in the field of vehicle image recognition and detection, can solve the problems of long training time, paralysis of the classifier training process, insufficient memory, etc., and achieve the effect of optimizing the training process
- Summary
- Abstract
- Description
- Claims
- Application Information
AI Technical Summary
Problems solved by technology
Method used
Image
Examples
Embodiment 1
[0030] please see Figure 1 to Figure 4 In this embodiment, 2000 vehicle positive sample pictures and 5000 vehicle negative sample pictures are manually selected, and the vehicle positive sample pictures and vehicle negative sample pictures are normalized to a picture of 64×64 pixels, wherein, the vehicle positive sample picture The selection standard is to include the left and right sides of the vehicle and expand 10% left and right, and include all images under the front car and expand 10% downward; the vehicle negative sample image is a road or natural scene image without a vehicle.
[0031] After normalizing the positive and negative vehicle images, the integral channel features are used to characterize the positive and negative images to form feature vectors.
[0032] The integral channel feature trains the positive and negative sample pictures with a picture size of 64×64 pixels, and speeds up the training by reducing the trained picture by a certain number of times. The...
Embodiment 2
[0065] This embodiment is the same as Embodiment 1 except for the following features: After normalizing the vehicle positive sample picture and the vehicle negative sample picture, HOG features are used to characterize the normalized positive and negative sample pictures to form features.
[0066] In this characterization process, the number of occurrences of local orientation gradients in positive and negative sample images will be counted. In the positive and negative sample images, the appearance and shape of local objects can be well described by the direction density distribution of gradients or edges. Therefore, the positive and negative sample pictures are divided into small connected regions (cell units), and then the gradient or edge direction histogram of each pixel in the cell unit is collected, and finally these histograms are combined to form a feature descriptor. The steps are as follows:
[0067] 1) Preprocess the positive and negative sample images, and then c...
PUM
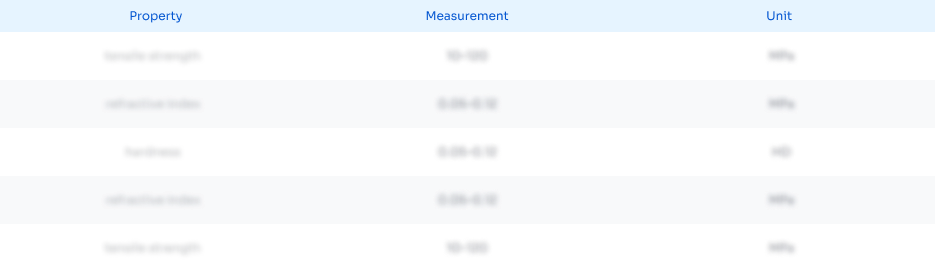
Abstract
Description
Claims
Application Information
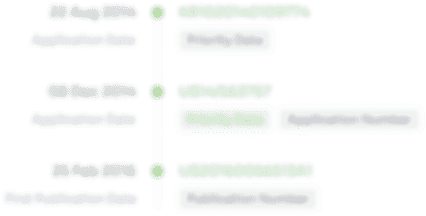
- R&D Engineer
- R&D Manager
- IP Professional
- Industry Leading Data Capabilities
- Powerful AI technology
- Patent DNA Extraction
Browse by: Latest US Patents, China's latest patents, Technical Efficacy Thesaurus, Application Domain, Technology Topic, Popular Technical Reports.
© 2024 PatSnap. All rights reserved.Legal|Privacy policy|Modern Slavery Act Transparency Statement|Sitemap|About US| Contact US: help@patsnap.com