Speech recognition method
A speech recognition and feature parameter technology, applied in speech recognition, speech analysis, instruments, etc., can solve the problems of heavy tailing feature optimization, inability to retain the inherent nonlinear structure and characteristics of high-dimensional data, etc., and achieve excellent dimensionality reduction effect Effect
- Summary
- Abstract
- Description
- Claims
- Application Information
AI Technical Summary
Problems solved by technology
Method used
Image
Examples
Embodiment 1
[0036] In the absence of feature optimization, speech recognition includes two steps: feature extraction and recognition using a classifier.
[0037] 1. Feature extraction:
[0038] The existing feature parameter MFCC and the feature parameter GCWT of the present invention are respectively extracted for speech.
[0039] 1. Feature parameter MFCC extraction steps:
[0040] (1) After the signal S(n) is pre-emphasized, the Hamming window is used for windowing and framing, and the signal x of each frame is obtained n (m), and then get its spectrum X by short-time Fourier transform n (k), then find the square of the spectrum, that is, the energy spectrum P n (k).
[0041] P n (k)=|X n (k)| 2
[0042] (2) Use M Mel bandpass filters to P n (k) Perform filtering, since the effects of the components in each frequency band are superimposed in the human ear, so the energy in each filter frequency band is superimposed.
[0043] S n ( ...
Embodiment 2
[0058] A speech recognition method includes three steps of feature extraction, feature optimization and recognition using a classifier.
[0059] 1. Feature extraction The steps of feature parameter GCWT extraction in Embodiment 1 are the same.
[0060] 2. Feature optimization:
[0061] Using the nonlinear dimensionality reduction method LLE for dimensionality reduction processing includes the following three steps:
[0062] (1) For a given source data set X={x 1 ,x 2 ,...,x n},x i ∈R D , using the Euclidean distance to find the
[0063] k (k
[0064] (2) Calculate the local reconstruction weight matrix of the sample point from the neighboring points of the sample point to minimize the reconstruction error;
[0065] (3) Calculate the low-dimensional embedding of the sample set according to the local reconstruction weight matrix and its neighbor points.
[0066] LLE uses the Euclidean distance to find the neighborhood under the sample unif...
Embodiment 3
[0070] A speech recognition method includes three steps of feature extraction, feature optimization and recognition using a classifier.
[0071] 1. Feature extraction The steps for extracting feature parameters GCWT in Embodiment 1 are the same.
[0072] 2. Feature optimization:
[0073] GCWT is dimensionally reduced using the nonlinear dimensionality reduction method DWLLE. During dimensionality reduction, the parameters are set as follows: k = 7 , σ = 1 7 , θ = 0.8 . The main process includes:
[0074] (1) Use Euclidean distance to find k (k<n) neighbor points of each sample point;
[0075] (2) Calculate the radial basis kernel function between the sample point and the neighbor point:
[0076] u ij = k ( x i , x j ) ...
PUM
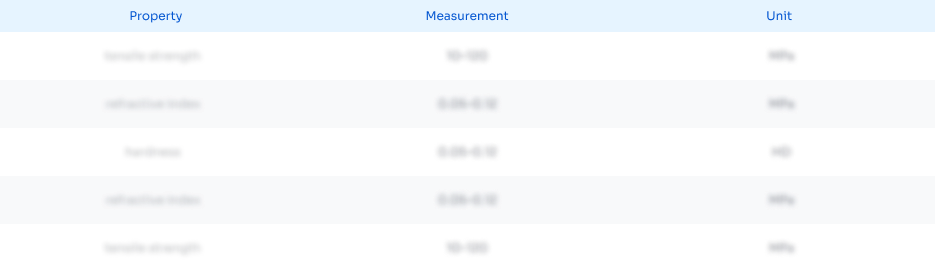
Abstract
Description
Claims
Application Information
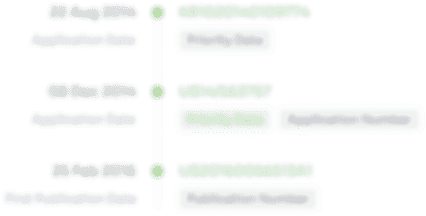
- Generate Ideas
- Intellectual Property
- Life Sciences
- Materials
- Tech Scout
- Unparalleled Data Quality
- Higher Quality Content
- 60% Fewer Hallucinations
Browse by: Latest US Patents, China's latest patents, Technical Efficacy Thesaurus, Application Domain, Technology Topic, Popular Technical Reports.
© 2025 PatSnap. All rights reserved.Legal|Privacy policy|Modern Slavery Act Transparency Statement|Sitemap|About US| Contact US: help@patsnap.com