Nonlinear characteristic extraction and classification based on defect data
A technology with nonlinear features and missing data. It is used in character and pattern recognition, instruments, computer parts, etc., and can solve problems such as the effect of manifold learning algorithms.
- Summary
- Abstract
- Description
- Claims
- Application Information
AI Technical Summary
Problems solved by technology
Method used
Image
Examples
Embodiment Construction
[0061] see figure 1 , a kind of non-linear feature extraction and classification method based on defective data provided by the present invention, comprises the following steps:
[0062] Step 101: Using a distance estimation method for missing data to construct a local neighborhood set of data points and a missing marker set corresponding to the local neighborhood set.
[0063] For each data point x i (i=1,2,…,n), define a missing marker vector f i =(f i1 ,f i2 ,..., f im ) T , where f it = 0 if and only if data point x i The tth attribute value in is missing, otherwise f it =1, then the entire data set X=(x 1 ,x 2 ,…,n) the missing marker matrix is F=(f 1 ,f 2 ,..., f n ). The present invention is based on the basic idea of cosine similarity, regards data points as their corresponding vectors, then two data points x with missing values i and x j The distance between can be expressed as
[0064] s i m ( ...
PUM
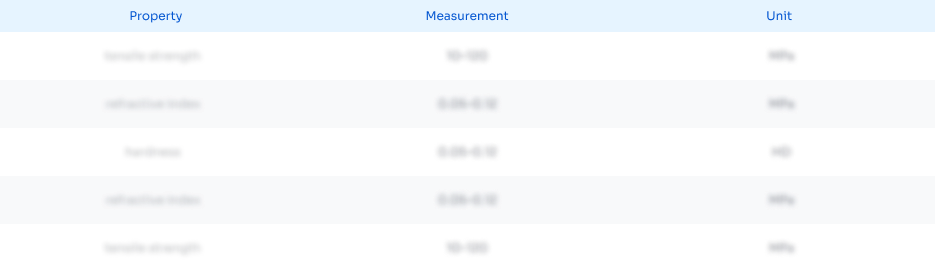
Abstract
Description
Claims
Application Information
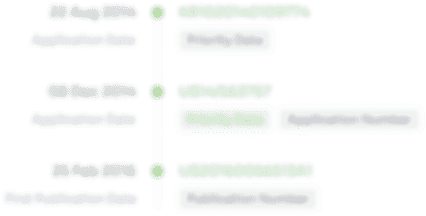
- R&D Engineer
- R&D Manager
- IP Professional
- Industry Leading Data Capabilities
- Powerful AI technology
- Patent DNA Extraction
Browse by: Latest US Patents, China's latest patents, Technical Efficacy Thesaurus, Application Domain, Technology Topic, Popular Technical Reports.
© 2024 PatSnap. All rights reserved.Legal|Privacy policy|Modern Slavery Act Transparency Statement|Sitemap|About US| Contact US: help@patsnap.com