Smiling face synthesis method based on segment-type sparse component analysis model
A sparse component analysis and synthesis method technology, applied in the field of smiley face synthesis, can solve the problems of complex face modeling models, high computational complexity, and lack of robustness
- Summary
- Abstract
- Description
- Claims
- Application Information
AI Technical Summary
Problems solved by technology
Method used
Image
Examples
Embodiment 1
[0060] Embodiment 1: A method for synthesizing a smiley face based on a partial sparse component analysis model, which is implemented through the following steps:
[0061] Step 1. Learn to build a generative model for face representation.
[0062] The generative model finds a common spatial segmentation for a given face image and learns a sparse component analysis model for each part of this segmentation. Suppose the probability distribution of the production model is P(x, Z, R), and its calculation method is P(x, Z, R) = P(x|R, Z)P(R)P(Z). First, derive the continuous induction prior of the production model, and use PoE (product ofexperts) to combine the continuous induction prior and the polynomial prior to form P(R), namely:
[0063] P ( R ) = Π d ...
Embodiment 2
[0091] Embodiment 2; A kind of smiling face synthesis method based on the partial sparse component analysis model, concrete implementation steps are described in detail as follows (using Visual C++ language programming to realize):
[0092] 1. Learn the face part prior, and realize the partial representation of the face.
[0093] In this embodiment, the CBCL face database is used to learn the face part prior, and it is transferred to the smile synthesis experiment on the face and facial expression database. The number of face parts is set to K=6, and the number of bases is set to M=40. M works well over a large range (empirically M ∈ [20, 240]) and is able to learn reasonable priors on face parts. The basis learned by the face part is shown in Figure 2. For comparison, Figure 2 also lists the bases learned by the other two methods. These two methods are Multiple Cause Factor Analysis (MCFA) ) and Structured Sparse Principle Component Analysis (SSPCA). Among Fig. 2, Fig. 2 (...
PUM
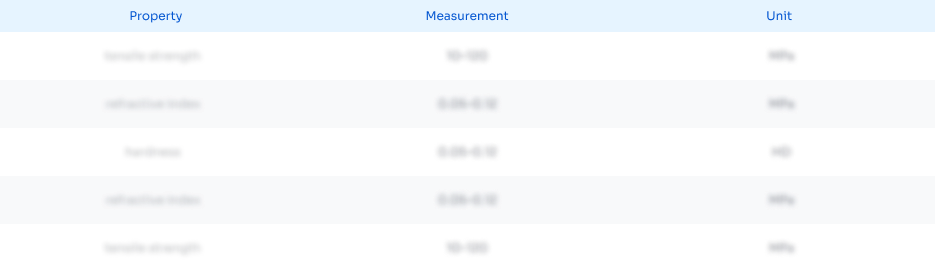
Abstract
Description
Claims
Application Information
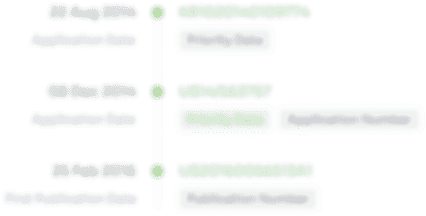
- R&D Engineer
- R&D Manager
- IP Professional
- Industry Leading Data Capabilities
- Powerful AI technology
- Patent DNA Extraction
Browse by: Latest US Patents, China's latest patents, Technical Efficacy Thesaurus, Application Domain, Technology Topic, Popular Technical Reports.
© 2024 PatSnap. All rights reserved.Legal|Privacy policy|Modern Slavery Act Transparency Statement|Sitemap|About US| Contact US: help@patsnap.com