A Classifier Design Method Based on Self-Explanatory Sparse Representations in Kernel Space
A sparse representation and design method technology, applied in the field of pattern recognition, can solve the problems of large fitting errors and low accuracy of classifiers, and achieve the effect of reducing fitting errors and improving performance
- Summary
- Abstract
- Description
- Claims
- Application Information
AI Technical Summary
Problems solved by technology
Method used
Image
Examples
Embodiment Construction
[0056] The present invention will be further described below in conjunction with a simulation example and in conjunction with the accompanying drawings.
[0057] A classifier design method based on sparse representation in a classification set based on kernel space, comprising the following steps:
[0058] Step 1: Design a classifier, the steps are:
[0059] (1) Read the training samples, the training samples have a total of C classes, define X=[X 1 ,X 2 ,...,X c ,...,X C ]∈R D×N Indicates the training samples, D is the face feature dimension, N is the total number of training samples, X 1 ,X 2 ,...,X c ,...,X C respectively represent the 1st, 2nd,...,c,...,C class samples, define N 1 ,N 2 ,...,N c ,...,N C Respectively represent the number of training samples of each type, then N=N 1 +N 2 +…+N c +…+N C ;
[0060] (2) Carry out two-norm normalization to the training samples to obtain normalized training samples;
[0061] (3) Take out each class in the trainin...
PUM
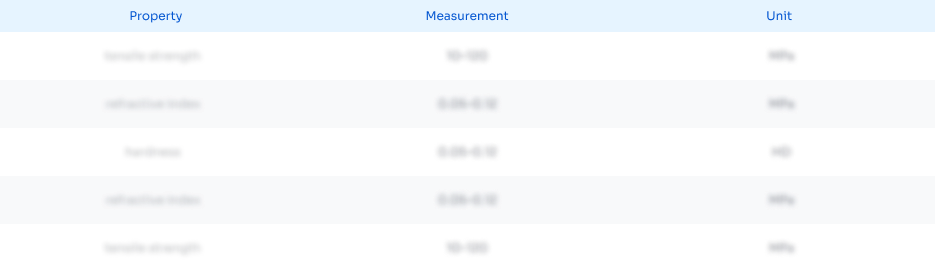
Abstract
Description
Claims
Application Information
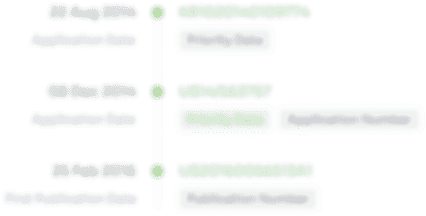
- R&D Engineer
- R&D Manager
- IP Professional
- Industry Leading Data Capabilities
- Powerful AI technology
- Patent DNA Extraction
Browse by: Latest US Patents, China's latest patents, Technical Efficacy Thesaurus, Application Domain, Technology Topic, Popular Technical Reports.
© 2024 PatSnap. All rights reserved.Legal|Privacy policy|Modern Slavery Act Transparency Statement|Sitemap|About US| Contact US: help@patsnap.com