Multi-instance multi-label learning based area monitoring method used in wireless sensor network
A wireless sensor network, multi-label learning technology, applied in network topology, wireless communication, data exchange network and other directions, can solve problems such as mass sensor data compression
- Summary
- Abstract
- Description
- Claims
- Application Information
AI Technical Summary
Problems solved by technology
Method used
Image
Examples
Embodiment Construction
[0064] The technical solutions of the present invention will be described in detail below in conjunction with the accompanying drawings.
[0065] Such as figure 1 The overall flowchart of the present invention is shown, and the present invention includes three processes: data collection, model training / updating, and network state prediction.
[0066] First, it is necessary to train a prediction model through the collected data, such as figure 2 As shown, the steps are as follows:
[0067] Step 1: Collect sensor network data at T moments to form a data set D o ={(N t ,Y t ), t=1,2,...,T}, N t ={s t,1 ,s t,2 ,...,S t,i ,...,S t,M} represents the data collected by the sensor network at time t, s t,i Indicates the data collected by the i-th sensor at time t, M is the total number of sensors in the entire sensor network, Y t ={y t,1 ,y t,2 ,...,y t,l ,...,y t,L} represents the state vector of the sensor network at time t, Y t There are L states y that need to be mo...
PUM
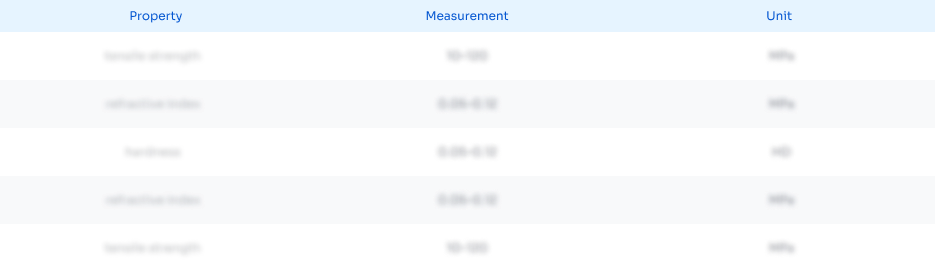
Abstract
Description
Claims
Application Information
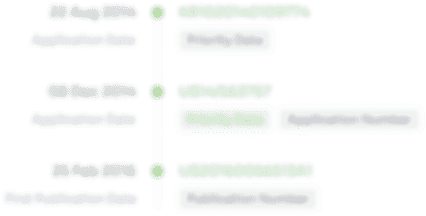
- R&D
- Intellectual Property
- Life Sciences
- Materials
- Tech Scout
- Unparalleled Data Quality
- Higher Quality Content
- 60% Fewer Hallucinations
Browse by: Latest US Patents, China's latest patents, Technical Efficacy Thesaurus, Application Domain, Technology Topic, Popular Technical Reports.
© 2025 PatSnap. All rights reserved.Legal|Privacy policy|Modern Slavery Act Transparency Statement|Sitemap|About US| Contact US: help@patsnap.com