Nerve network clustering method based on iteration
A neural network and clustering algorithm technology, applied in the field of iterative neural network clustering, can solve problems such as long running time, large memory, high-dimensional linearity in data space, etc.
- Summary
- Abstract
- Description
- Claims
- Application Information
AI Technical Summary
Problems solved by technology
Method used
Image
Examples
Embodiment Construction
[0044] The present invention will be further described below in combination with specific embodiments.
[0045] Specify a sample set to be clustered And the number of categories K to be clustered, where d represents the feature dimension of the sample, N represents the number of samples, and x i Is a d-dimensional feature vector, that is, a matrix with 1 row and d columns. Here we take the Iris classic data set in UCI as an example to be clustered. The number of categories K to be clustered is 3, the feature dimension d is 4, and the number of samples N is 150. x i is a matrix with 1 row and 4 columns.
[0046]First, in step 1, the task of initializing the necessary parameters of the ELM must be completed. Step 1 includes the following two sub-steps:
[0047] Step 1.1, set the number L of neurons in the hidden layer of the ELM model, the activation function g(θ) of the hidden layer, and the regularization term coefficient γ. The value of the number L of neurons in the hid...
PUM
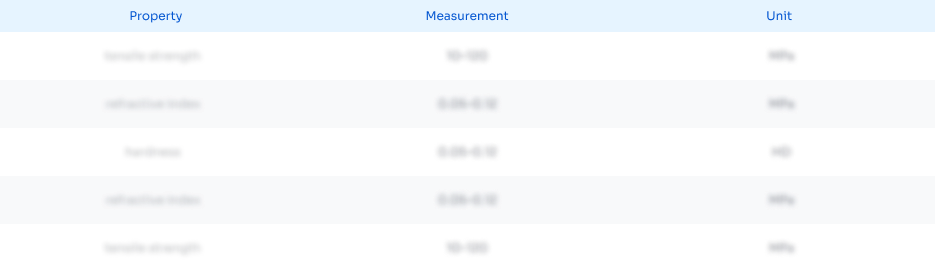
Abstract
Description
Claims
Application Information
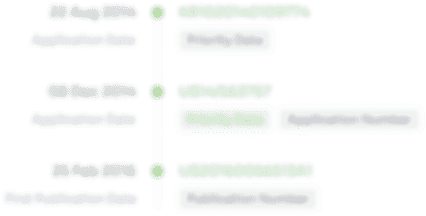
- R&D Engineer
- R&D Manager
- IP Professional
- Industry Leading Data Capabilities
- Powerful AI technology
- Patent DNA Extraction
Browse by: Latest US Patents, China's latest patents, Technical Efficacy Thesaurus, Application Domain, Technology Topic, Popular Technical Reports.
© 2024 PatSnap. All rights reserved.Legal|Privacy policy|Modern Slavery Act Transparency Statement|Sitemap|About US| Contact US: help@patsnap.com