Chemical process fault monitoring method based on active learning
A chemical process and fault monitoring technology, applied in program control, electrical test/monitoring, test/monitoring control systems, etc., can solve problems such as difficult to collect fault type data and limit the application of naive Bayesian classifiers
- Summary
- Abstract
- Description
- Claims
- Application Information
AI Technical Summary
Problems solved by technology
Method used
Image
Examples
Embodiment Construction
[0048] The specific implementation manners of the present invention will be described in detail below in conjunction with the accompanying drawings.
[0049] Such as figure 1 As shown, the chemical process fault monitoring method based on active learning of the present embodiment is applied in the TennesseeEastmanProcess (TEP) process; the TEP industrial process is created by Eastman Chemical Company of the United States, and this industrial process has four reactants (A, C , D, E), produce two products (G and H), wherein, material A is H 2 Hydrogen, material B is N 2 Ammonia, material C is CO carbon monoxide, material D is CH 3 OH methanol, material E is C 2 h 5 OH ethanol, product G is C 2 h 6 o 2 Ethylene glycol, product H is C 3 h 8 o 2 Propylene glycol; the whole process includes five main reaction devices: reactor, condenser, cycle compressor, desorption tower and gas-liquid separator, with a total of 50 variables and 16 failure types.
[0050] A chemical proc...
PUM
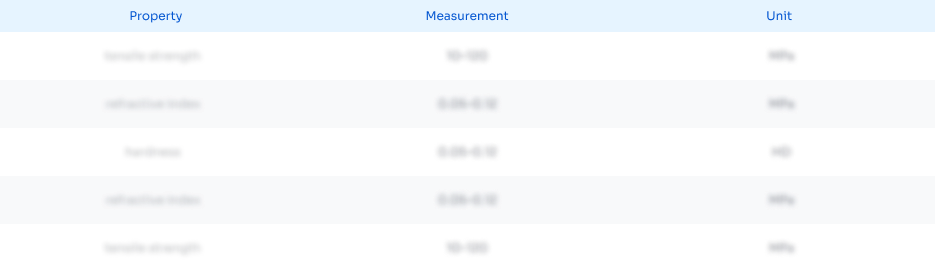
Abstract
Description
Claims
Application Information
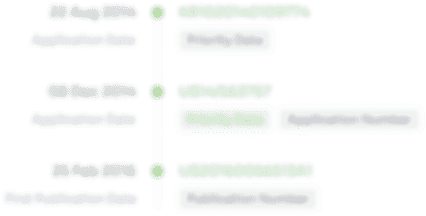
- R&D Engineer
- R&D Manager
- IP Professional
- Industry Leading Data Capabilities
- Powerful AI technology
- Patent DNA Extraction
Browse by: Latest US Patents, China's latest patents, Technical Efficacy Thesaurus, Application Domain, Technology Topic, Popular Technical Reports.
© 2024 PatSnap. All rights reserved.Legal|Privacy policy|Modern Slavery Act Transparency Statement|Sitemap|About US| Contact US: help@patsnap.com