Object segmentation method based on multiple-instance learning and graph cuts optimization
A multi-instance learning and target segmentation technology, applied in the field of image processing, can solve the problems of the lack of learning ability of the model, the inability to reflect the visual attention mechanism well, and the lack of image adaptability.
- Summary
- Abstract
- Description
- Claims
- Application Information
AI Technical Summary
Problems solved by technology
Method used
Image
Examples
Embodiment Construction
[0047] Such as figure 1 As shown, the object segmentation method based on multi-instance learning and graph cut optimization of the present invention specifically includes the following steps:
[0048] Step 1: Use the method of multi-instance learning to model the saliency model on the training image, and use the saliency model to predict the packages and examples in the test image, and obtain the saliency detection result of the test image;
[0049] Step 2: Introduce the saliency of the test image into the graph cut framework, optimize the graph cut framework according to the example feature vector and the label of the example package, use the agglomerative hierarchical clustering algorithm to solve the suboptimal solution of the graph cut optimization, and obtain the accurate segmentation of the target .
[0050] Further, said step 1 includes step 11 and step 12:
[0051] Step 11, preprocessing the training image, and extracting image brightness gradient features and color...
PUM
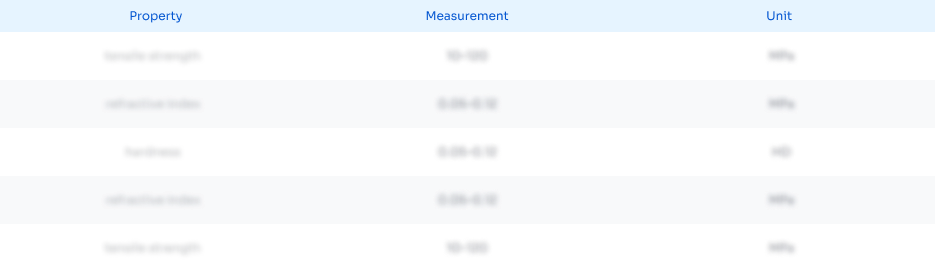
Abstract
Description
Claims
Application Information
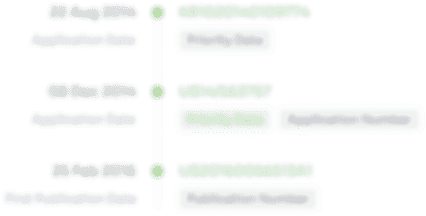
- R&D Engineer
- R&D Manager
- IP Professional
- Industry Leading Data Capabilities
- Powerful AI technology
- Patent DNA Extraction
Browse by: Latest US Patents, China's latest patents, Technical Efficacy Thesaurus, Application Domain, Technology Topic, Popular Technical Reports.
© 2024 PatSnap. All rights reserved.Legal|Privacy policy|Modern Slavery Act Transparency Statement|Sitemap|About US| Contact US: help@patsnap.com