Hyperspectral Image Classification Method Based on Ridgelet and Deep Convolutional Networks
A hyperspectral image, deep convolution technology, applied in the field of hyperspectral image classification, can solve the problems of small computational complexity, difficult to achieve, difficult to learn effective classification features, etc., to improve the classification accuracy and speed of classification. Effect
- Summary
- Abstract
- Description
- Claims
- Application Information
AI Technical Summary
Problems solved by technology
Method used
Image
Examples
Embodiment Construction
[0027] The technical solutions and effects of the present invention will be described in further detail below with reference to the accompanying drawings.
[0028] refer to figure 1 , the implementation steps of the present invention are as follows:
[0029] Step 1, input image.
[0030] Input a hyperspectral image, as shown in the figure, where 2(a) is the input hyperspectral image, figure 2 (b) is the class label image corresponding to 2(a), and 10% of the pixels from 2(a) are selected as training samples.
[0031] Step 2, extract the spectral information of the training samples.
[0032] Assuming that the spectral dimension of the hyperspectral image input in step 1 is V, for each training sample, extract the spectral value of each dimension of the sample to form a spectral vector f j ,j=1,...,J, J is the number of training samples, spectral vector f j The dimension of is V.
[0033] Step 3, reduce the dimension of the hyperspectral image.
[0034] The methods for i...
PUM
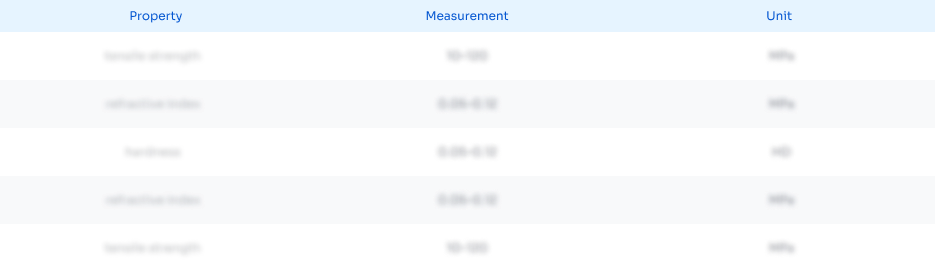
Abstract
Description
Claims
Application Information
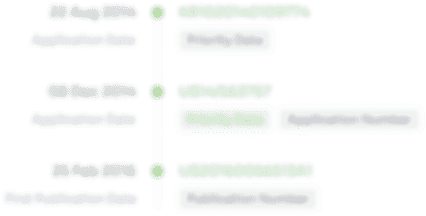
- Generate Ideas
- Intellectual Property
- Life Sciences
- Materials
- Tech Scout
- Unparalleled Data Quality
- Higher Quality Content
- 60% Fewer Hallucinations
Browse by: Latest US Patents, China's latest patents, Technical Efficacy Thesaurus, Application Domain, Technology Topic, Popular Technical Reports.
© 2025 PatSnap. All rights reserved.Legal|Privacy policy|Modern Slavery Act Transparency Statement|Sitemap|About US| Contact US: help@patsnap.com