Multi-subject fMRI data analysis method combining ICA and shift invariant CPD
A data analysis and multi-subject technology, applied in the field of medical signal processing, can solve problems such as easy divergence of iterative operations, no consideration of TC differences, no objective function, etc.
- Summary
- Abstract
- Description
- Claims
- Application Information
AI Technical Summary
Problems solved by technology
Method used
Image
Examples
Embodiment Construction
[0030] A specific embodiment of the present invention will be described in detail below in conjunction with the technical scheme and accompanying drawings.
[0031] There are 16 fMRI data collected under the finger-tapping task, that is, K=16. Each subject underwent J=165 scans, each scan obtained 53×63×46 whole brain data, and the number of voxels in the brain I=59610. Assuming that 16 subjects share SM and share TC component number N=35, the steps of fMRI data analysis using the present invention are shown in the attached figure.
[0032] Step 1: Input multi-subject fMRI data k=1,...,16,
[0033] Step 2: Perform two-stage PCA compression. The first level PCA compresses each subject data from 165×59610 to 50×59610, namely N 1 =50; the second-level PCA compresses the time-dimension series data of 16 subjects from 800×59610 to 35×59610; obtains the compressed matrix
[0034] The third step: ICA preprocessing. The fastICA algorithm was used to perform ICA on 35×59610 ...
PUM
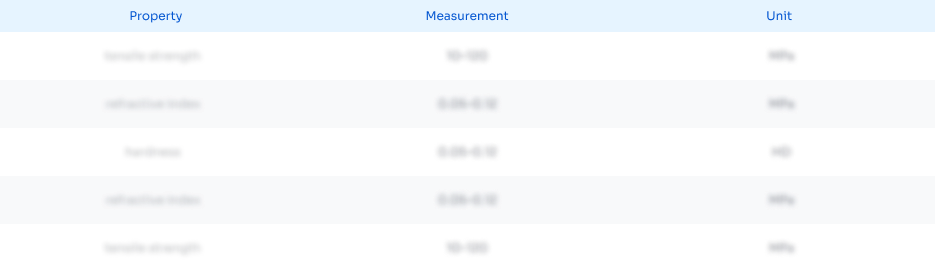
Abstract
Description
Claims
Application Information
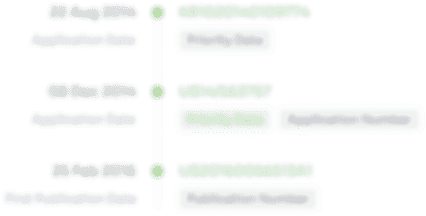
- R&D
- Intellectual Property
- Life Sciences
- Materials
- Tech Scout
- Unparalleled Data Quality
- Higher Quality Content
- 60% Fewer Hallucinations
Browse by: Latest US Patents, China's latest patents, Technical Efficacy Thesaurus, Application Domain, Technology Topic, Popular Technical Reports.
© 2025 PatSnap. All rights reserved.Legal|Privacy policy|Modern Slavery Act Transparency Statement|Sitemap|About US| Contact US: help@patsnap.com