Behavior recognition method based on sparse spatial-temporal characteristics
A technology of spatio-temporal features and recognition methods, applied in character and pattern recognition, instruments, computer components, etc., can solve problems such as inability to guarantee the optimal solution, and achieve the effect of improving behavior recognition rate, performance and performance
- Summary
- Abstract
- Description
- Claims
- Application Information
AI Technical Summary
Problems solved by technology
Method used
Image
Examples
Embodiment 1
[0030] Embodiment one: see figure 1 As shown, a behavior recognition method based on sparse spatio-temporal features includes the following steps:
[0031] Step 1. For the input video, use spatio-temporal Gabor to convolve with the original input video to construct a scale space;
[0032] Step 2. Use the expressions of different scales as the values of different channels of the spatio-temporal deep belief network, and jointly learn multi-scale features;
[0033] Step 3: Identify and classify behavioral features.
[0034] In the first step, considering the complexity of model training, from the representations of 7 different scales, according to the loss of information between representations of different scales, based on the entropy, 3 scales with the smallest loss are selected as the multi-scale representation of the input video, Input the deep model for multi-scale feature learning.
[0035] In this embodiment, the Gabor function is used to fit the receptive field respo...
Embodiment 2
[0069] Embodiment two: the behavior database used in this embodiment is KTH (Kungliga Tekniska h?gskolan, Royal Institute of Technology, Sweden), including six types of behaviors: boxing (boxing), clapping (handclapping), waving (handwaving), jogging (jogging) , running (running) and walking (walking), each behavior was repeated multiple times by 25 actors in four different environments. Nine actors in the dataset (actors 2, 3, 5, 6, 7, 8, 9, 10 and 22) form the test set, and the remaining 16 actors are equally divided into training and validation sets. Experimental hardware environment: Linux, Intel(R) Xeon(R) CPU E5-2620 v22.1GHz, 62.9G memory, 1T hard disk. The code running environment is: MATLAB 2013a.
[0070] see image 3 As shown, the motion information of the boxing behavior on KTH at different scales is given, and each column corresponds to a different frame in the video. It can be seen from the figure that with the scale (here used Representation) keeps getting b...
PUM
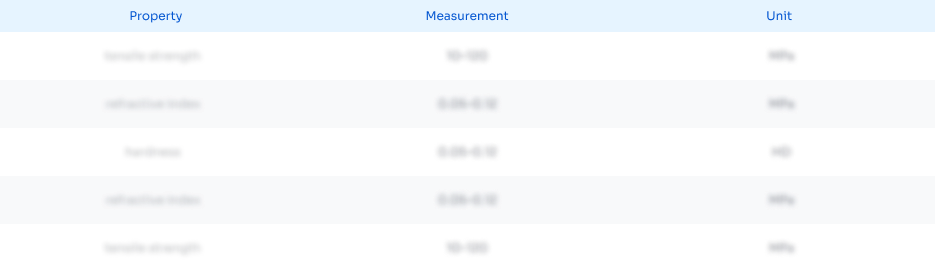
Abstract
Description
Claims
Application Information
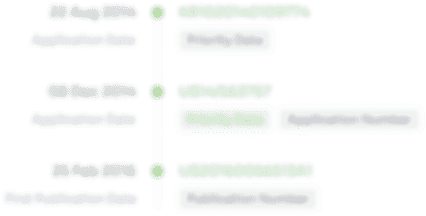
- Generate Ideas
- Intellectual Property
- Life Sciences
- Materials
- Tech Scout
- Unparalleled Data Quality
- Higher Quality Content
- 60% Fewer Hallucinations
Browse by: Latest US Patents, China's latest patents, Technical Efficacy Thesaurus, Application Domain, Technology Topic, Popular Technical Reports.
© 2025 PatSnap. All rights reserved.Legal|Privacy policy|Modern Slavery Act Transparency Statement|Sitemap|About US| Contact US: help@patsnap.com