Multi-spectrum data supervised classification method based on relevant linear information entropy
A supervised classification and linear correlation technology, applied in the field of remote sensing data interpretation, to achieve accurate classification results and efficient calculations
- Summary
- Abstract
- Description
- Claims
- Application Information
AI Technical Summary
Problems solved by technology
Method used
Image
Examples
specific Embodiment approach 1
[0031] Specific implementation mode one: as figure 1 As shown, the multispectral data supervised classification method combined with linear correlation information entropy provided in this embodiment is divided into three steps, and the specific steps are as follows:
[0032] Step 1: Automatically screen the training sample set required for supervised classification after manual sampling.
[0033] 1) For the captured multispectral remote sensing images Among them, Row and Column represent the width and length of the multispectral remote sensing image, and B represents the number of bands of the multispectral remote sensing image. The image analyst selects the training samples according to the sampler of F×F size. In order to ensure that the subsequent screening work can be carried out, F is required ≥2, that is, F can be selected at one time 2 pixels of training samples, with a vector P m , m=1,...,F 2 to represent that each P m The dimensions of are equal to B, represen...
specific Embodiment approach 2
[0070] Embodiment 2: In this embodiment, a standard multi-spectral image data, FLCl data set is selected. This data is the airborne 12-band multispectral data taken in June 1966 in a certain area in the south of Tippecanoe County, Indiana, USA, and the ground truth reference map was given by the relevant scientific research personnel on the spot. Therefore, using this data set for experiments can be Practical evaluation of the classification accuracy of the classifier algorithm. The configuration of the computer used in the experiment is as follows: Intel i32.3GHz processor, 4G memory.
PUM
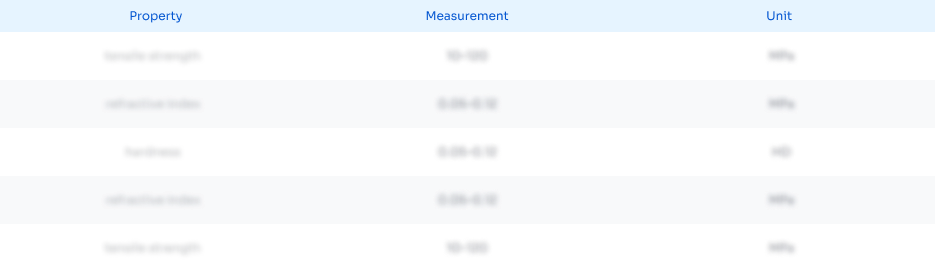
Abstract
Description
Claims
Application Information
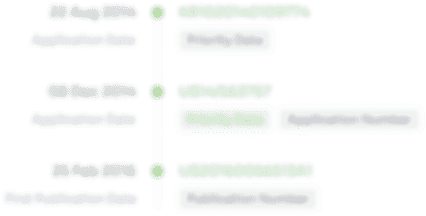
- R&D Engineer
- R&D Manager
- IP Professional
- Industry Leading Data Capabilities
- Powerful AI technology
- Patent DNA Extraction
Browse by: Latest US Patents, China's latest patents, Technical Efficacy Thesaurus, Application Domain, Technology Topic, Popular Technical Reports.
© 2024 PatSnap. All rights reserved.Legal|Privacy policy|Modern Slavery Act Transparency Statement|Sitemap|About US| Contact US: help@patsnap.com