A Nonconvex Compressive Sensing Image Reconstruction Method Based on Local Similarity and Local Selection
A compressed sensing and image reconstruction technology, applied in the field of image processing, which can solve the problems of inaccurate image reconstruction, good visual effect, and high peak signal-to-noise ratio.
- Summary
- Abstract
- Description
- Claims
- Application Information
AI Technical Summary
Problems solved by technology
Method used
Image
Examples
Embodiment 1
[0064] Embodiment 1, with reference to figure 1 A detailed description.
[0065] The invention is a non-convex compressive sensing image reconstruction method based on local similarity and local selection. The method can perform low-speed sampling and a small amount of sampling on the image signal, and then reconstruct the image accurately, which greatly reduces the storage limit and calculation of the device. The specific implementation steps are as follows:
[0066] Step (1), observe and receive the original image after segmentation.
[0067] Input the original image and divide it into 16*16 non-overlapping blocks, use the random Gaussian observation matrix Φ to observe each block to obtain the measurement vector y, the sending end sends the observation matrix Φ and the measurement vector y of each block, and the receiving end receives it;
[0068] In the present embodiment, the image of 512 * 512 is divided into image blocks of 16 * 16 to obtain 1024 image blocks; all ima...
Embodiment 2
[0075] Embodiment 2, in conjunction with attached figure 1 -6 Description.
[0076] On the basis of Example 1, the step (2) uses the local similarity of the standard deviation of the observed vectors to cluster the observed vectors of all image blocks using a local growth method, specifically including the following steps:
[0077] 2.1) Calculate the standard deviation of each observation vector.
[0078] 2.2) Set a clustering mark for all image blocks, initially all marks are 0, where mark 0 means not clustered, and mark 1 means included in a certain category.
[0079] 2.3) Starting from the first image block, perform the following operations on each image block in turn: if the image block clustering mark is 1, no operation is performed; if the mark is 0, perform the Mth i clustering of classes.
[0080] Carry out the Mth i The specific steps of class clustering are as follows:
[0081] In the first step, the current image block i is used as the seed image block, and the...
PUM
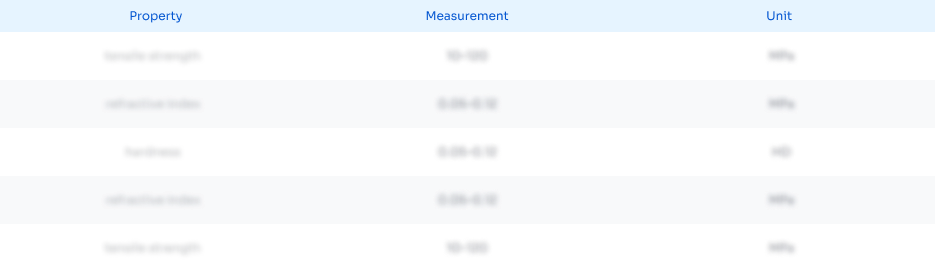
Abstract
Description
Claims
Application Information
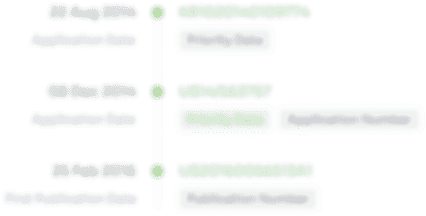
- R&D
- Intellectual Property
- Life Sciences
- Materials
- Tech Scout
- Unparalleled Data Quality
- Higher Quality Content
- 60% Fewer Hallucinations
Browse by: Latest US Patents, China's latest patents, Technical Efficacy Thesaurus, Application Domain, Technology Topic, Popular Technical Reports.
© 2025 PatSnap. All rights reserved.Legal|Privacy policy|Modern Slavery Act Transparency Statement|Sitemap|About US| Contact US: help@patsnap.com