Low-rank constraint online self-supervised learning scene classification method
A technique of supervised learning and low-rank constraints, which is applied in the direction of instruments, character and pattern recognition, computer components, etc., and can solve the problem that the measurement matrix is susceptible to noise and interference
- Summary
- Abstract
- Description
- Claims
- Application Information
AI Technical Summary
Problems solved by technology
Method used
Image
Examples
Embodiment Construction
[0034] The present invention will be further described in detail below in conjunction with the accompanying drawings and embodiments.
[0035] To achieve classification based on our online metric learning model, we define a bilinear graphical model to predict the label of the next new test sample, fusing information from labeled and unlabeled data in a semi-supervised learning manner. Then design a unified online self-updating model framework to handle online scene classification, such as figure 1 shown.
[0036] We propose an online framework such as figure 1 As shown, metric learning is used to measure similarity and semi-supervised learning is used to label test samples. The specific method is to overcome the over-fitting computer automatic scene classification method through low-rank constraints, provide a matrix model to focus on adaptive similarity learners, and establish an algorithm framework based on image analysis and machine learning.
[0037] Such as figure 2 ...
PUM
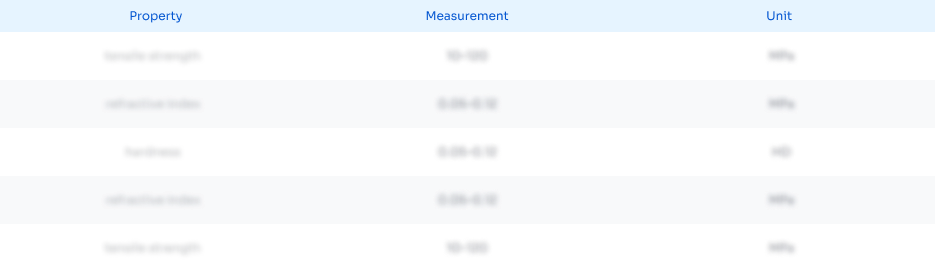
Abstract
Description
Claims
Application Information
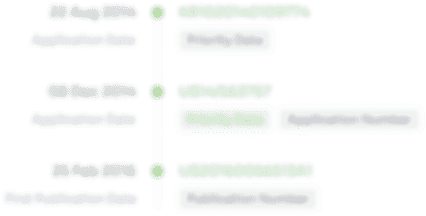
- R&D Engineer
- R&D Manager
- IP Professional
- Industry Leading Data Capabilities
- Powerful AI technology
- Patent DNA Extraction
Browse by: Latest US Patents, China's latest patents, Technical Efficacy Thesaurus, Application Domain, Technology Topic, Popular Technical Reports.
© 2024 PatSnap. All rights reserved.Legal|Privacy policy|Modern Slavery Act Transparency Statement|Sitemap|About US| Contact US: help@patsnap.com