Intelligent fault diagnosis method based on rough Bayesian network classifier
A Bayesian network and fault diagnosis technology, applied in the field of electromechanical, can solve the problems of reducing the scale of the Bayesian network model, shortening the calculation time of Bayesian network inference, low efficiency, etc., to overcome the problem of rule search and critical misjudgment , avoid the curse of dimensionality problem, reduce the effect of scale
- Summary
- Abstract
- Description
- Claims
- Application Information
AI Technical Summary
Problems solved by technology
Method used
Image
Examples
Embodiment Construction
[0032] The present invention will be further described below in conjunction with the accompanying drawings and embodiments.
[0033] 1. Collect characteristic data and construct fault diagnosis information decision table based on rough set
[0034] The invention takes a complex rotor system as an example to diagnose the fault. According to the collected fault characteristic data, the original fault diagnosis information decision table T can be established 1 As shown in Table 1, the seven conditional attributes are: C 1 Indicates 0.01~0.40f, C 2 Indicates 0.41~0.50f, C 3 Indicates 0.51~0.99f, C 4 means 1f, C 5 Indicates 2f, C 6 Indicates 3~5f, C 7 Indicates 5f; the five fault types are: D 1 Indicates that the rotor is unbalanced, D 2 Indicates that the rotor is misaligned, D 3 Indicates oil film oscillation, D 4 Indicates surge, D 5 Indicates a collision.
[0035] Table 1 Decision table of original fault diagnosis information of a certain rotor system
[0036] ...
PUM
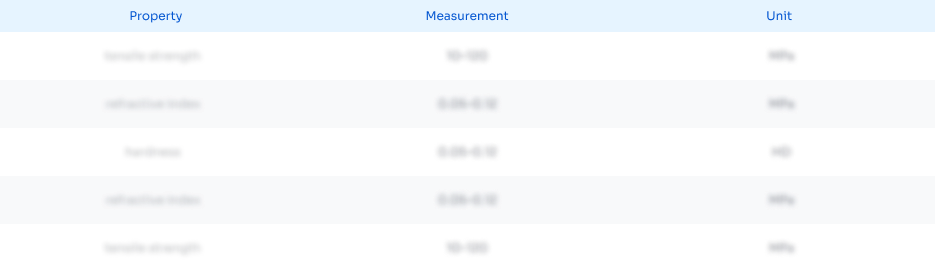
Abstract
Description
Claims
Application Information
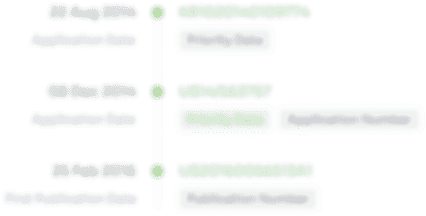
- Generate Ideas
- Intellectual Property
- Life Sciences
- Materials
- Tech Scout
- Unparalleled Data Quality
- Higher Quality Content
- 60% Fewer Hallucinations
Browse by: Latest US Patents, China's latest patents, Technical Efficacy Thesaurus, Application Domain, Technology Topic, Popular Technical Reports.
© 2025 PatSnap. All rights reserved.Legal|Privacy policy|Modern Slavery Act Transparency Statement|Sitemap|About US| Contact US: help@patsnap.com