Recommending method integrating user and project rating and characteristic factors
A feature factor and recommendation method technology, applied in the field of recommendation research, can solve problems such as cold start, limited item features, and over-rating
- Summary
- Abstract
- Description
- Claims
- Application Information
AI Technical Summary
Problems solved by technology
Method used
Image
Examples
Embodiment Construction
[0040] The specific steps of the user recommendation result constructed by the present invention are as follows:
[0041] Step 1: Construct a user-based and user-based similarity model SimUser 1 (i, j), SimUser 2 (i,j) are as follows:
[0042] SimUser 1 ( i , j ) = Σ c ∈ I i , j ( R i , c - A i ) ( R ...
PUM
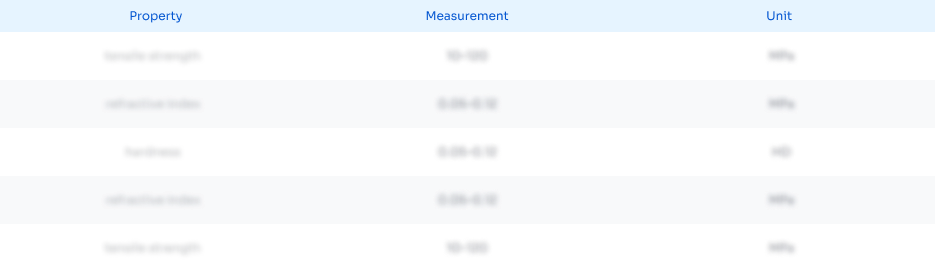
Abstract
Description
Claims
Application Information
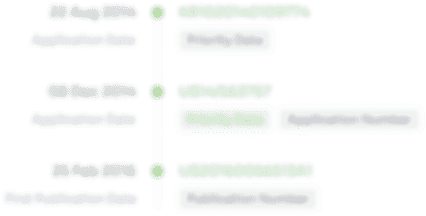
- R&D Engineer
- R&D Manager
- IP Professional
- Industry Leading Data Capabilities
- Powerful AI technology
- Patent DNA Extraction
Browse by: Latest US Patents, China's latest patents, Technical Efficacy Thesaurus, Application Domain, Technology Topic, Popular Technical Reports.
© 2024 PatSnap. All rights reserved.Legal|Privacy policy|Modern Slavery Act Transparency Statement|Sitemap|About US| Contact US: help@patsnap.com