Feedback blind equalization method of dynamic wavelet neural network based on fuzzy control
A technology of fuzzy neural network and dynamic wavelet, which is applied to the shaping network in the transmitter/receiver, baseband system components, etc., can solve the problems of not adapting to time-varying characteristics, slow learning convergence speed, and sensitivity to external noise
- Summary
- Abstract
- Description
- Claims
- Application Information
AI Technical Summary
Problems solved by technology
Method used
Image
Examples
Embodiment 1
[0177] Example 1: Minimum Phase Underwater Acoustic Channel Simulation
[0178] The minimum phase underwater acoustic channel, its transfer function is:
[0179] c=[0.9656 -0.0906 0.0578 0.2368]
[0180] The transmitted signal is 4QAM, the signal-to-noise ratio is 20dB, the superimposed noise is Gaussian white noise, the WNN equalizer, the weight length is 16, and the eighth tap is initialized to 1. In formula (8), C WNN =10, λ in formula (14) WNN =0.46, the initialization of wavelet function scale factor and translation factor are respectively a WNN =4.3,b WNN =0.0025; For FDWNN equalizer, the weight length is 16, the 8th tap is 1, the weight length of the transversal filter is 16, 1 / 4 tap is used, the step size μ=0.001, the initialization of wavelet function scale factor and translation factor respectively a FDWNN =3,b FDWNN =0.006, the two weighting factors in the formula (12) are respectively α=0.355, β=0.645, C in the formula (8) FDWNN =1, λ in formula (14) FDWNN ...
Embodiment 2
[0182] Embodiment 2: Sparse underwater acoustic channel simulation
[0183] The transfer function of the sparse underwater acoustic channel used in this experiment is
[0184] c=zeros(1,1001); c(1)=0.076; c(2)=0.122; c(1001)=1
[0185]The transmitted signal is 8PSK, the signal-to-noise ratio is 20dB, the superimposed noise is Gaussian white noise, WNN equalizer, the weight length is 16, the sixth tap is initialized to 1, C in formula (8) WNN =0.28, λ in formula (14) WNN =5.3, the initialization of wavelet function scale factor and translation factor are respectively a WNN =5.2,b WNN =1.685; FDWNN equalizer, weight length is 16, adopts 1 / 2 tap, the weight length of transversal filter is 16, adopts 1 / 4 tap, step size μ=0.0001, the initialization of wavelet function scale factor and translation factor are respectively a FDWNN =4.35,b FDWNN =0.007, the two weighting factors in the formula (12) are respectively α=0.9075, β=0.0925, C in the formula (8) FDWNN =0.0001, λ in for...
PUM
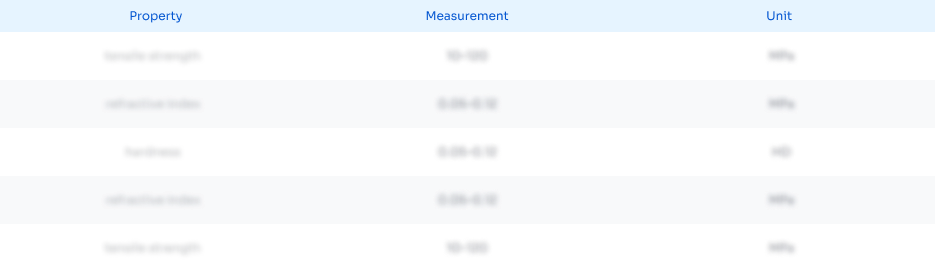
Abstract
Description
Claims
Application Information
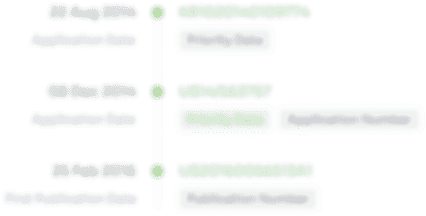
- Generate Ideas
- Intellectual Property
- Life Sciences
- Materials
- Tech Scout
- Unparalleled Data Quality
- Higher Quality Content
- 60% Fewer Hallucinations
Browse by: Latest US Patents, China's latest patents, Technical Efficacy Thesaurus, Application Domain, Technology Topic, Popular Technical Reports.
© 2025 PatSnap. All rights reserved.Legal|Privacy policy|Modern Slavery Act Transparency Statement|Sitemap|About US| Contact US: help@patsnap.com