Constraint heteroscedasticity linear discriminant analysis method for language identification
A linear discriminant analysis and language recognition technology, applied in speech analysis, speech recognition, instruments, etc.
- Summary
- Abstract
- Description
- Claims
- Application Information
AI Technical Summary
Problems solved by technology
Method used
Image
Examples
Embodiment Construction
[0052] Assuming that c is a supervector feature, for the HLDA algorithm, its goal is to find a linear transformation matrix A such that
[0053] x=Ac
[0054] In order to obtain a new vector after transformation, the discriminative information is compressed to the first several dimensions. In the present invention, c is spliced by the basic features, because DCT (discrete cosine transform) has been carried out when the basic features are obtained, the correlation in the basic feature frame is already weak, and the key programming of the problem removes the basic feature between frames relevance. Going back to the cepstral matrix C, we can only study the lateral transformation, and the corresponding transformation matrix A should have the form of a diagonal block matrix, namely
[0055]
[0056]In addition, according to the characteristics of supervector features, we can prove that its covariance matrix has the form of an approximate diagonal block matrix through theoret...
PUM
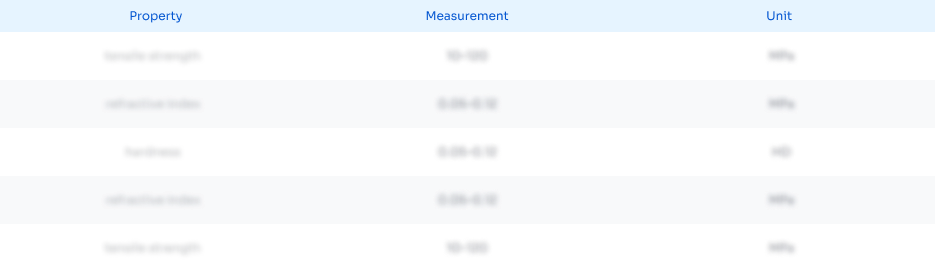
Abstract
Description
Claims
Application Information
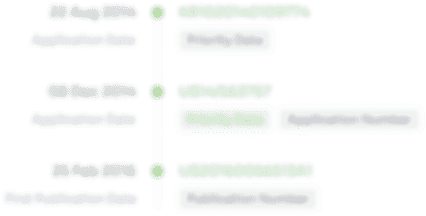
- R&D Engineer
- R&D Manager
- IP Professional
- Industry Leading Data Capabilities
- Powerful AI technology
- Patent DNA Extraction
Browse by: Latest US Patents, China's latest patents, Technical Efficacy Thesaurus, Application Domain, Technology Topic, Popular Technical Reports.
© 2024 PatSnap. All rights reserved.Legal|Privacy policy|Modern Slavery Act Transparency Statement|Sitemap|About US| Contact US: help@patsnap.com