High-order logic knowledge graph representation learning method based on structural features
A technology of high-level logic and knowledge graph, applied in the field of artificial intelligence research, can solve the problems of low computing cost, large representation loss, poor interpretability, etc., and achieve the effect of reducing computing cost and reducing the number of parameters
- Summary
- Abstract
- Description
- Claims
- Application Information
AI Technical Summary
Problems solved by technology
Method used
Image
Examples
Embodiment Construction
[0052] The specific embodiments of the present invention will be further described below with reference to the accompanying drawings and technical solutions.
[0053] A high-order logic knowledge graph representation learning method based on structural features, including three steps. First, perform high-order logical relationship feature representation on the data, extract the node neighborhood structure motif degree matrix in the graph, and use the entity motif degree matrix in each relationship as a high-order logical relationship feature; secondly, perform feature representation, the present invention The proposed knowledge graph representation learning method can consider both entity attribute features and higher-order logical relationship features. At each relation, the present invention uses a graph convolutional network to obtain representations of higher-order logical relational features and attribute features. Finally, feature aggregation is performed. The present i...
PUM
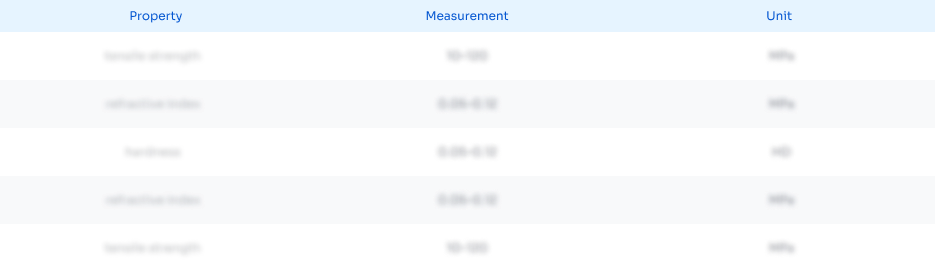
Abstract
Description
Claims
Application Information
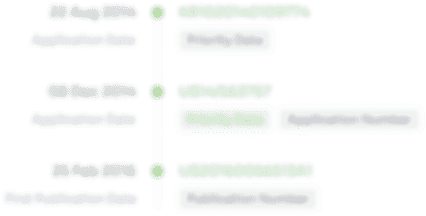
- R&D Engineer
- R&D Manager
- IP Professional
- Industry Leading Data Capabilities
- Powerful AI technology
- Patent DNA Extraction
Browse by: Latest US Patents, China's latest patents, Technical Efficacy Thesaurus, Application Domain, Technology Topic, Popular Technical Reports.
© 2024 PatSnap. All rights reserved.Legal|Privacy policy|Modern Slavery Act Transparency Statement|Sitemap|About US| Contact US: help@patsnap.com