Seismic reservoir prediction method based on ResNet
A technology for reservoir prediction and seismic data, applied in seismic signal processing and other directions, can solve the problems of weak learning ability, easy to fall into local minimum points, low prediction accuracy, etc., to reduce risks, have good application prospects, and improve reservoir prediction. The effect of precision
- Summary
- Abstract
- Description
- Claims
- Application Information
AI Technical Summary
Problems solved by technology
Method used
Image
Examples
Embodiment 1
[0029] like figure 1 As described, the seismic reservoir prediction method based on this embodiment (hereinafter referred to as the method of this embodiment) includes the following steps:
[0030] (1) Obtain the seismic data of the target interval in the work area, and extract the seismic amplitude data of the target interval from the seismic data of the target interval;
[0031] (2) Normalize the seismic amplitude data of the target interval to the [-1,1] interval, and input the normalized seismic amplitude data of the target interval into the pre-trained amplitude-sandbody thickness model to predict Obtain the sand body thickness of the target interval and complete the reservoir prediction.
[0032] Among them, the training process of the amplitude-sand body thickness model is as follows figure 2 shown, as follows:
[0033] (1) Obtain the logging data and the seismic data of the bypass channel of a plurality of known wells drilling into the target interval of the work a...
Embodiment 2
[0060] The difference between the ResNet-based seismic reservoir prediction method in this embodiment and the ResNet-based seismic reservoir prediction method in Method Embodiment 1 is only that: in this embodiment, the seismic amplitude data of the target interval is input into the pre-trained In the amplitude-porosity model of , the porosity of the target interval is predicted to complete the reservoir prediction.
[0061] Among them, the amplitude-porosity model is obtained through the following steps:
[0062] Obtain logging data and side-channel seismic data of multiple known wells drilling into the target interval of the work area;
[0063] The porosity at the target interval of each known well is calculated using the logging data of each known well, and the porosity at the target interval of each known well is extracted from the seismic data of the bypass channel of each known well. seismic amplitude data;
[0064] Take the seismic amplitude data at each known well ta...
Embodiment 3
[0066] The difference between the ResNet-based seismic reservoir prediction method in this embodiment and the ResNet-based seismic reservoir prediction method in Method Embodiment 1 is only that: in this embodiment, the pre-training is performed by inputting the seismic amplitude data of the target interval. The sand body thickness of the target interval can be predicted from the amplitude-sandbody thickness model of The sand body thickness and porosity of the section are determined to complete the reservoir prediction.
[0067] The training process of the amplitude-sand body thickness model is shown in Method Embodiment 1, and the training process of the amplitude-porosity model is shown in Method Embodiment 2, which will not be repeated here.
PUM
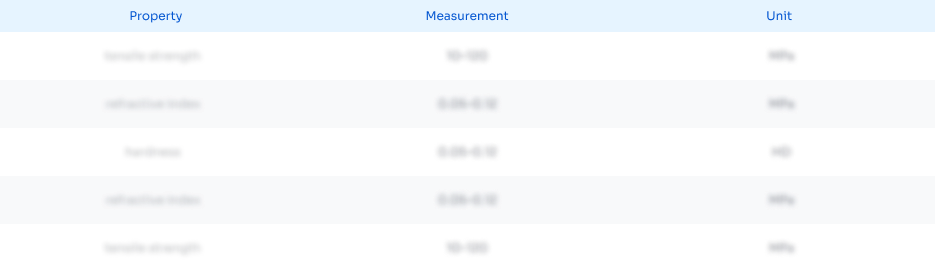
Abstract
Description
Claims
Application Information
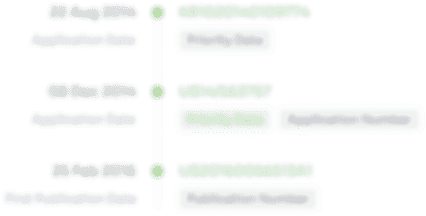
- R&D Engineer
- R&D Manager
- IP Professional
- Industry Leading Data Capabilities
- Powerful AI technology
- Patent DNA Extraction
Browse by: Latest US Patents, China's latest patents, Technical Efficacy Thesaurus, Application Domain, Technology Topic, Popular Technical Reports.
© 2024 PatSnap. All rights reserved.Legal|Privacy policy|Modern Slavery Act Transparency Statement|Sitemap|About US| Contact US: help@patsnap.com