Maximum cross-correlation entropy Kalman filtering method based on random weighting criterion
A criterion, the largest technology, applied in the field of signal processing, can solve the problem of the degradation of estimation performance, and achieve the effect of increasing the correlation entropy, improving the accuracy and the effectiveness of the estimation, and improving the accuracy.
- Summary
- Abstract
- Description
- Claims
- Application Information
AI Technical Summary
Problems solved by technology
Method used
Image
Examples
Embodiment 1
[0099] Consider a general linear system model:
[0100]
[0101]
[0102] Where θ = π / 18, and the system noise is Gaussian noise Q i (k-1) ~ n (0, 2) (i = 1, 2), the amount of measuring noise is a mixed Gaussian noise R (K) to 0.9N (0, 1) + 0.1 N (0,100).
[0103] According to the maximum correlation entropy Kalman filtering method based on the random weighted criterion, the state initial value is taken Error covariance matrix P (0 | 0) = DIAG (100,100). The nuclear width σ of the Gaussian nuclear function is set to 0.1, 0.5, 1, 2, 3, 5, 8, 10, compared to the KF algorithm and MCKF (σ = 2), respectively. figure 2 , 3 Two state x 1 , X 2 Probability density function under different filtering algorithms. The simulation results of the maximum correlation entropy Karman filtering method based on random weight weighted criteria are shown in the curve RWMCKF. It can be seen that more conventional KF and MCKF, the performance advantage is obvious: the KF algorithm is biased in the...
Embodiment 2
[0106] Replacing the simulation model is a one-dimensional line sex accelerated motion, the system model and measurement model are as follows:
[0107]
[0108]
[0109] Among them, Δt = 0.1s, and the system noise and quantitative noise are nonlinear mixed Gaussian noise:
[0110] Qi 1 (k-1) ~ 0.9N (0,0.01) + 0.1n (0, 1)
[0111] Qi 2 (k-1) ~ 0.9N (0,0.01) + 0.1n (0, 1)
[0112] Qi 3(k-1) ~ 0.9N (0,0.01) + 0.1n (0, 1)
[0113] R (k) ~ 0.8 N (0, 0.01) + 0.2N (0,100).
[0114] According to the maximum correlation entropy Kalman filtering method based on the random weighted criterion, the filtering algorithm is first initialized. State initial value and covariance at the beginning value are set to x (0) = [001] T , P (0 | 0) = DIAG (0.01, 0.01, 0.01). The nuclear width σ of the Gaussian nuclear function is set to 2 (which is relatively optimum in Example 1), and the mean square error under KF, MCKF (σ = 2) and RWMCKF (σ = 2) algorithm can be obtained. surface:
[0115] Table I KF...
PUM
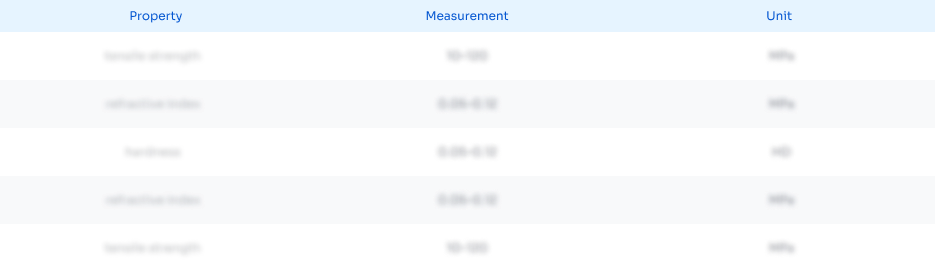
Abstract
Description
Claims
Application Information
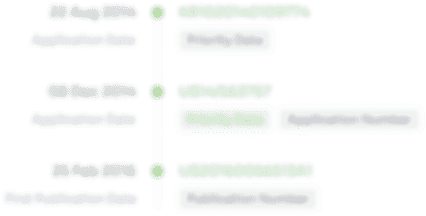
- R&D Engineer
- R&D Manager
- IP Professional
- Industry Leading Data Capabilities
- Powerful AI technology
- Patent DNA Extraction
Browse by: Latest US Patents, China's latest patents, Technical Efficacy Thesaurus, Application Domain, Technology Topic, Popular Technical Reports.
© 2024 PatSnap. All rights reserved.Legal|Privacy policy|Modern Slavery Act Transparency Statement|Sitemap|About US| Contact US: help@patsnap.com