Transformer winding fault diagnosis method based on improved G-means vector element
A transformer winding and fault diagnosis technology, applied in the direction of instruments, character and pattern recognition, calculation models, etc., can solve the problems of signal over-decomposition, insufficient decomposition, interference signal components, etc., to improve classification accuracy and fault diagnosis accuracy Effect
- Summary
- Abstract
- Description
- Claims
- Application Information
AI Technical Summary
Problems solved by technology
Method used
Image
Examples
Embodiment Construction
[0043] The following is a detailed description of the improved G-means vector element transformer winding fault diagnosis method of the present invention.
[0044] A transformer winding fault diagnosis method with improved G-means vector element, comprising the following steps:
[0045] 1. Collect vibration signals of transformer windings, perform G-means vector element decomposition VED on the actually measured vibration signals of transformer windings, introduce deviation coefficient γ, and obtain K deviation vector functions IMγ. The deviation vector function corrected by the deviation coefficient has better Obitoker stability, which is beneficial to participate in the calculation of the subsequent G-means vector element results optimized by the artificial sardine swarm algorithm.
[0046] 2. Construct signal feature vector (energy entropy and root mean square value)
[0047] The concept of VED energy entropy is introduced: the VED decomposition of the vibration signal of ...
PUM
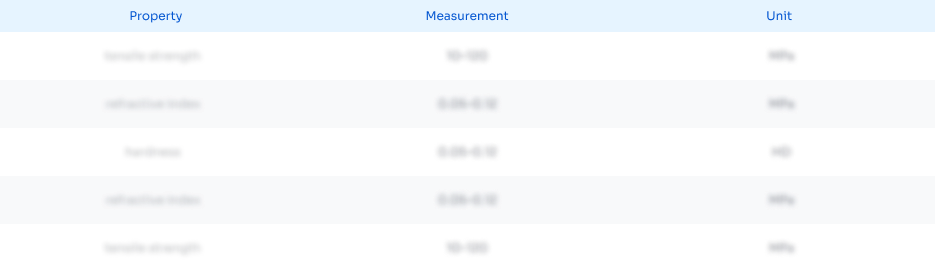
Abstract
Description
Claims
Application Information
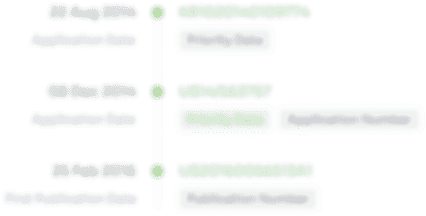
- R&D Engineer
- R&D Manager
- IP Professional
- Industry Leading Data Capabilities
- Powerful AI technology
- Patent DNA Extraction
Browse by: Latest US Patents, China's latest patents, Technical Efficacy Thesaurus, Application Domain, Technology Topic, Popular Technical Reports.
© 2024 PatSnap. All rights reserved.Legal|Privacy policy|Modern Slavery Act Transparency Statement|Sitemap|About US| Contact US: help@patsnap.com