Training method and device of detection segmentation model, and target detection method and device
A technology for segmentation models and training methods, applied in neural learning methods, biological neural network models, image analysis, etc., to achieve the effect of improving segmentation accuracy and reducing quantity requirements
- Summary
- Abstract
- Description
- Claims
- Application Information
AI Technical Summary
Problems solved by technology
Method used
Image
Examples
Embodiment 1
[0107] see figure 2 as shown, figure 2 It is a schematic flowchart of training a pair of detection and segmentation models in an embodiment. This training method comprises, the first stage of execution step 201 and the second stage of execution step 202, wherein:
[0108] Step 201 , using pixel-level labeled sample data for training with a detection and segmentation task to obtain a detection and segmentation model with first model parameters.
[0109] see image 3 as shown, image 3 It is a schematic diagram of a framework for training a detection and segmentation model using pixel-level labeled sample data in Embodiment 1. The backbone network is connected with a detection segmentation model.
[0110] Step 2011, when the pixel-level labeled sample data is input to the backbone network, for example, a frame of pixel-level labeled sample image data is input to the backbone network, and the backbone network extracts the features in the pixel-level labeled sample data to ...
Embodiment 2
[0146] see Figure 5 as shown, Figure 5 It is a schematic flow chart of training the detection and segmentation model in the second embodiment. This training method comprises, the first stage of execution step 501 and the second stage of execution step 502, wherein:
[0147] Step 501 , use pixel-level labeled sample data to conduct multi-task training including detection and segmentation tasks and target classification tasks to obtain a detection and segmentation model with the first model parameters and an image classification model with the third model parameters.
[0148] see Figure 6 as shown, Figure 6 A schematic diagram of a framework for training a detection segmentation model using pixel-level labeled sample data. The backbone network is connected with image classification model and detection segmentation model in parallel. Step 5011, when the pixel-level labeled sample data is input to the backbone network, for example, a frame of pixel-level labeled sample im...
PUM
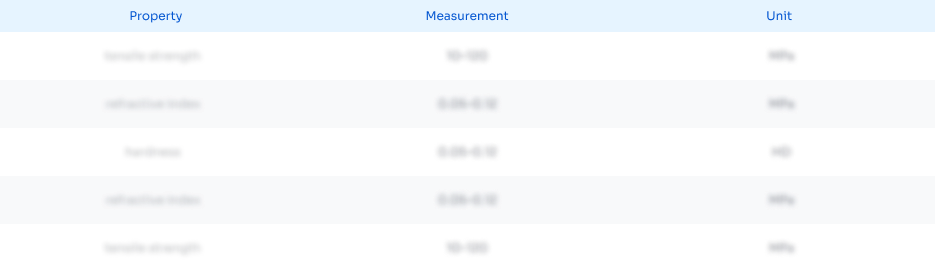
Abstract
Description
Claims
Application Information
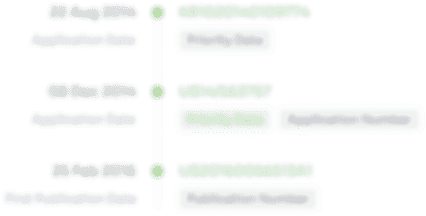
- R&D Engineer
- R&D Manager
- IP Professional
- Industry Leading Data Capabilities
- Powerful AI technology
- Patent DNA Extraction
Browse by: Latest US Patents, China's latest patents, Technical Efficacy Thesaurus, Application Domain, Technology Topic, Popular Technical Reports.
© 2024 PatSnap. All rights reserved.Legal|Privacy policy|Modern Slavery Act Transparency Statement|Sitemap|About US| Contact US: help@patsnap.com