Recommendation method based on multi-behavior session graph fusion
A recommendation method and behavioral technology, applied in marketing, advertising, instruments, etc., can solve the problems of reducing the diversity of recommendation results, ignoring users' future behaviors, and difficult for models to acquire "novel" items, so as to improve diversity and The effect of accuracy
- Summary
- Abstract
- Description
- Claims
- Application Information
AI Technical Summary
Problems solved by technology
Method used
Image
Examples
Embodiment 1
[0034] In order to ensure high connectivity and item diversity of the sparse behavior session graph, preferably, the value of N in step b) is 10.
Embodiment 2
[0036] Step c) comprises the following steps:
[0037] c-1) Take joint session itemsets Items in the adjacency matrix A are initialized as graph nodes click , to traverse the click sequence of the target user and neighbor users, if item j is the next item clicked by the user after item i is clicked, then construct an edge between item i and item j, that is, the adjacency matrix A click The middle abscissa is the value of i and the ordinate is j Increase by 1, for the adjacency matrix A click Normalize to get the click session graph
[0038] c-2) Take joint session itemsets Items in the graph node initialize the collection adjacency matrix A collect , to traverse the collection sequence of the target user and neighbor users, if item j is the next favorite item after the user’s favorite item i, then construct an edge between item i and item j, that is, the adjacency matrix A collect The middle abscissa is the value of i and the ordinate is j Increase by 1, for the a...
Embodiment 3
[0041] In step d) through the formula Calculate the final embedding vector e obtained by the high-order propagation of act behavior i,act , act is one of the behaviors of click, collection and purchase, is the embedding vector of message aggregation of node i layer l in the act behavior session graph, where a l is the propagation weight, L is the total number of layers of propagation, L=2, is the l-layer neighbor node item set of node i in the act behavior session graph, for e i,act The weights of node i and neighbor node j in the corresponding adjacency matrix, for The embedding vector of node j in .
PUM
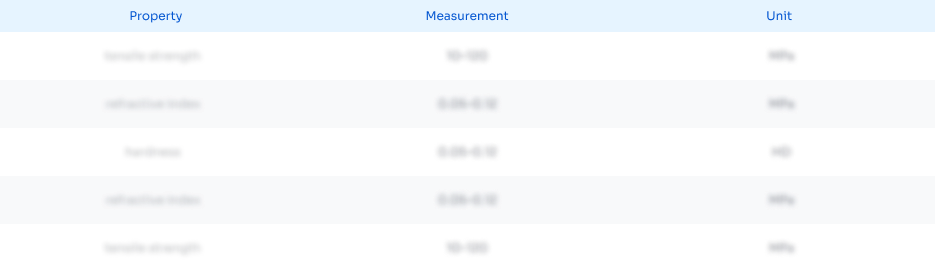
Abstract
Description
Claims
Application Information
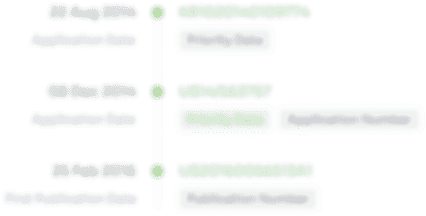
- R&D Engineer
- R&D Manager
- IP Professional
- Industry Leading Data Capabilities
- Powerful AI technology
- Patent DNA Extraction
Browse by: Latest US Patents, China's latest patents, Technical Efficacy Thesaurus, Application Domain, Technology Topic, Popular Technical Reports.
© 2024 PatSnap. All rights reserved.Legal|Privacy policy|Modern Slavery Act Transparency Statement|Sitemap|About US| Contact US: help@patsnap.com