Two-stage behavior recognition fine classification method based on graph convolutional network
A convolutional network and subdivision technology, applied in the field of two-stage behavior recognition subdivision, can solve the problem of not classifying highly similar actions well, and achieve the effect of expanding the receptive field and improving the accuracy rate
- Summary
- Abstract
- Description
- Claims
- Application Information
AI Technical Summary
Problems solved by technology
Method used
Image
Examples
Embodiment 1
[0054] One proposed based on the behavior of the present invention is to identify a two-stage network of FIG convolutional fine classification method, disclosed in the experimental data set NTU-RGB + D 60, and the method and the results were compared mainstream. According to mainstream practice, experiments were performed on X-Sub and X-View two benchmark, using Top1 as an evaluation index. This experiment was carried out using only a single embodiment of the data stream (as joint data), and the results compared with only a single stream model.
[0055] Experimental parameters of the invention to:
[0056] Experimental environment for the present invention: processor Intel (R) Xeon (R) CPU E5-2603 v4@1.70GHz, graphics card, an NVIDIA Titan XP 12GB, memory 64GB, operating system Ubuntu 16.04 (64-bit), the programming language Python3.7.4, deep learning framework for PyTorch1.2.0.
[0057] Training and testing process model, a continuous human skeletal joints 300 as input data, i.e....
PUM
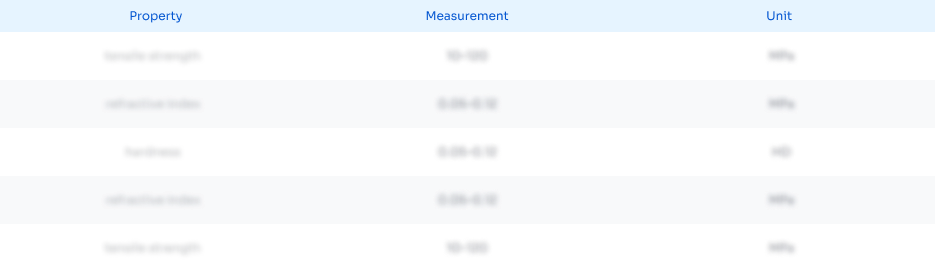
Abstract
Description
Claims
Application Information
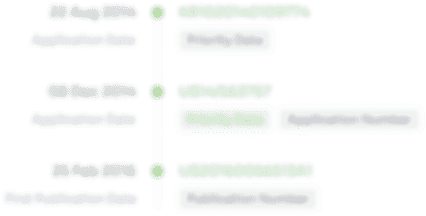
- R&D Engineer
- R&D Manager
- IP Professional
- Industry Leading Data Capabilities
- Powerful AI technology
- Patent DNA Extraction
Browse by: Latest US Patents, China's latest patents, Technical Efficacy Thesaurus, Application Domain, Technology Topic, Popular Technical Reports.
© 2024 PatSnap. All rights reserved.Legal|Privacy policy|Modern Slavery Act Transparency Statement|Sitemap|About US| Contact US: help@patsnap.com