Lake TN prediction method based on VMD-CSSA-LSTM-MLR combined model
A technology combining models and forecasting methods, applied in forecasting, neural learning methods, biological neural network models, etc., can solve problems such as difficulty in determining the hyperparameters of machine learning algorithms, differences in machine learning models, and poor data prediction capabilities, so as to improve the overall situation Search capability, improved efficiency and accuracy, and fast running effects
- Summary
- Abstract
- Description
- Claims
- Application Information
AI Technical Summary
Problems solved by technology
Method used
Image
Examples
Embodiment 1
[0084] A lake TN prediction method based on the VMD-CSSA-LSTM-MLR combination model, such as figure 1 shown, including the following steps:
[0085] Step 1, using empirical mode decomposition (EMD) to adaptively decompose the collected original TN data sequence signal to obtain the effective modal component number K, and perform variational modal decomposition (VMD) on the original signal according to the effective modal component number K, Decomposed into K eigenmode components;
[0086] Step 1.1: Calculate each modal component u using Hilbert transform for the original TN data sequence signal k The analytical signal of (t), thus obtaining its one-sided spectrum as:
[0087]
[0088] Among them, t represents the t-th moment, k represents the k-th mode, j represents the imaginary unit, and σ(t) represents the center frequency of the k-th mode at the t-time;
[0089] Step 1.2: Analyze the signal and the corresponding center frequency through each mode mixes the terms to...
Embodiment 2
[0139] Collect all TN time series data from June 18, 2018 to December 31, 2019 from the Poyang Lake Water Quality Automatic Monitoring Station (Duchang Station), such as Figure 4 It can be seen that the data show certain nonlinear and non-stationary characteristics.
[0140] Substituting the above data into the method in Example 1 according to the time series, the last 20% as the verification set and the first 80% as the training set, the obtained VMD decomposition results are as follows Figure 5 As shown (the reason for adding the parameter K of VMD decomposition to 7 (such as Figure 5 decomposed into 7 subcomponents), firstly, according to the EMD (empirical mode decomposition) adaptive decomposition result is 7 (this step is the same as figure 1 The flow chart is corresponding), and according to Figure 5 In the spectrogram of (b), it can be found that the center frequency overlap problem does not occur when it is decomposed into 7, indicating the rationality of choosi...
PUM
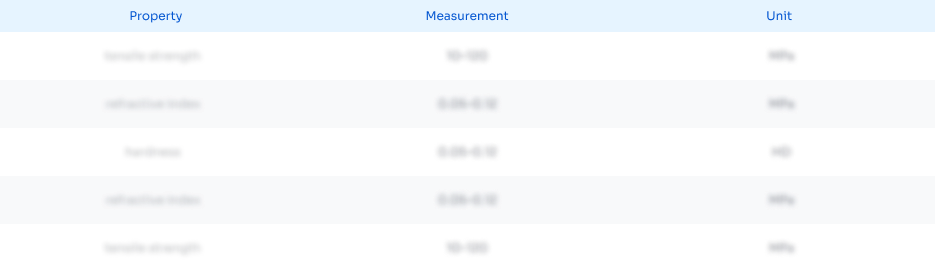
Abstract
Description
Claims
Application Information
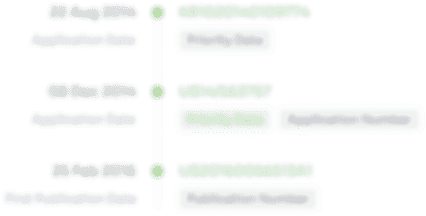
- R&D Engineer
- R&D Manager
- IP Professional
- Industry Leading Data Capabilities
- Powerful AI technology
- Patent DNA Extraction
Browse by: Latest US Patents, China's latest patents, Technical Efficacy Thesaurus, Application Domain, Technology Topic, Popular Technical Reports.
© 2024 PatSnap. All rights reserved.Legal|Privacy policy|Modern Slavery Act Transparency Statement|Sitemap|About US| Contact US: help@patsnap.com