Deep cluster neural network model construction method for pneumatic data processing
A neural network model, aerodynamic data technology, applied in biological neural network models, neural learning methods, electrical digital data processing, etc., to achieve the effects of improving prediction accuracy, improving learning accuracy, and accelerating convergence speed
- Summary
- Abstract
- Description
- Claims
- Application Information
AI Technical Summary
Problems solved by technology
Method used
Image
Examples
Embodiment 1
[0045] As a preferred embodiment of the present invention, it discloses a method for constructing a deep cluster neural network model for aerodynamic data processing, the steps of which are as follows:
[0046] a. Preparation and preprocessing of data sets: firstly, aerodynamic data sets are obtained through computational fluid dynamics methods, wind tunnel experiments or flight tests, and the main design parameters and response parameters in the aerodynamic data sets are extracted; then, according to the distribution characteristics of the data, the aerodynamic The data in the data set is classified and divided into multiple subsets; each subset is labeled to identify which cluster in the deep cluster neural network plays a role in the training of a certain subset, each in the deep cluster neural network The cluster corresponds to the data in the labeled subset; finally, all the data in the aerodynamic data set are normalized and divided into training set, validation set and ...
Embodiment 2
[0052] See attached figure 1 And attached figure 2 , another preferred embodiment of the present invention is: the present invention aims at the defects and deficiencies in the existing data acquisition methods in the field of aerodynamic data modeling, and proposes a method for modeling aerodynamic data distribution characteristics based on cluster neural An approach to aerodynamic modeling of networks. Based on the network structure of the cluster network, this method proposes a modeling method oriented to the distribution characteristics of aerodynamic data to meet the needs of aerodynamic data modeling. The cluster neural network trained by this method can make up for the defects of the traditional neural network model in the environment of insufficient sample quality caused by uneven sample sampling or uneven sample distribution when processing aerodynamic data, thereby improving the performance of the model. precision.
[0053] Proceed as follows:
[0054] a. Prepar...
Embodiment 3
[0076] See attached figure 1 And attached figure 2 , as the best implementation mode of the present invention is: in the step a, before classifying the data in the aerodynamic data set, there is also a data cleaning step: for the data in the aerodynamic data set, if there are outliers and null values, perform Remove processing. Therefore, the prediction accuracy of the constructed deep cluster neural network model is enhanced.
PUM
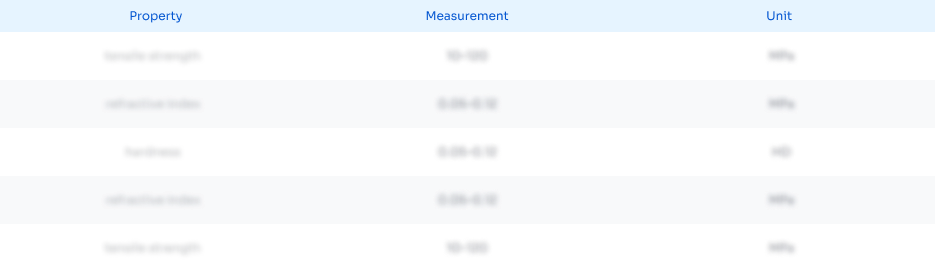
Abstract
Description
Claims
Application Information
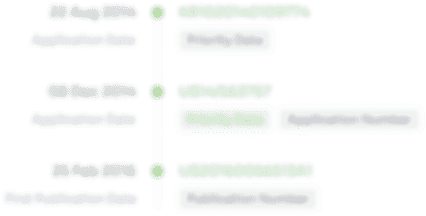
- R&D Engineer
- R&D Manager
- IP Professional
- Industry Leading Data Capabilities
- Powerful AI technology
- Patent DNA Extraction
Browse by: Latest US Patents, China's latest patents, Technical Efficacy Thesaurus, Application Domain, Technology Topic, Popular Technical Reports.
© 2024 PatSnap. All rights reserved.Legal|Privacy policy|Modern Slavery Act Transparency Statement|Sitemap|About US| Contact US: help@patsnap.com