Hashing-based effective user modeling
A user and hash code technology, applied in the field of user activity modeling and similarity search, can solve the problem of high computing cost
- Summary
- Abstract
- Description
- Claims
- Application Information
AI Technical Summary
Problems solved by technology
Method used
Image
Examples
Embodiment Construction
[0027] Hashing learning is widely adopted as a solution to approximate nearest neighbor search for large-scale data retrieval in a variety of applications. Applying deep architectures to learn hashing has particular benefits due to its computational efficiency and retrieval quality. However, these deep architectures may not be well-suited to correctly handle data known as "sequential behavior data". Sequential behavioral data may include data types observed in application scenarios related to user modeling. In certain embodiments, to learn a binary hash for sequential behavioral data, the system can capture users' evolving preferences (e.g., measured over extended time periods) or exploit user activity patterns on different time scales (e.g., , by comparing activity patterns on short and long timescales). The disclosed techniques provide novel deep learning-based architectures to learn binary hashes for sequential behavioral data. The effectiveness of the architecture of th...
PUM
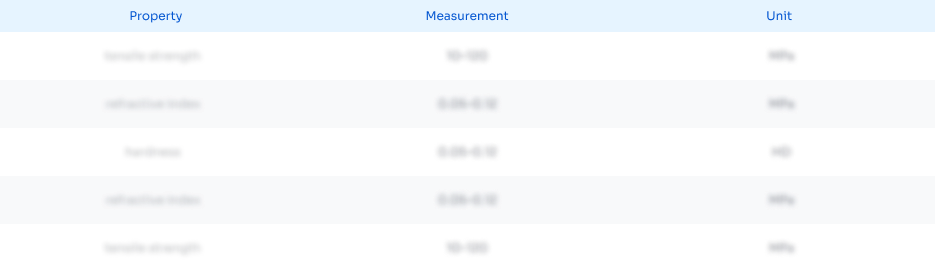
Abstract
Description
Claims
Application Information
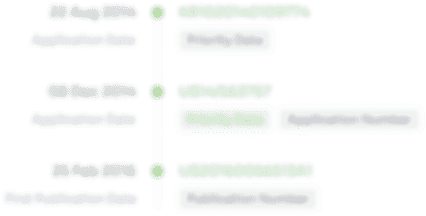
- R&D Engineer
- R&D Manager
- IP Professional
- Industry Leading Data Capabilities
- Powerful AI technology
- Patent DNA Extraction
Browse by: Latest US Patents, China's latest patents, Technical Efficacy Thesaurus, Application Domain, Technology Topic, Popular Technical Reports.
© 2024 PatSnap. All rights reserved.Legal|Privacy policy|Modern Slavery Act Transparency Statement|Sitemap|About US| Contact US: help@patsnap.com