Road monthly traffic volume prediction method based on SARIMA-NAR combined model
A technology combining models and forecasting methods, applied in traffic flow detection, road vehicle traffic control systems, neural learning methods, etc., can solve the problem that static neural networks have no memory function, less research on seasonal fluctuations, and low time series forecasting ability and other problems, to achieve the effect of convenient prediction process, high validity and reliability, and rich theoretical research system
- Summary
- Abstract
- Description
- Claims
- Application Information
AI Technical Summary
Problems solved by technology
Method used
Image
Examples
example
[0062] Example: A road monthly traffic forecast method based on SARIMA-NAR combined model, including the following steps:
[0063] 1) Collect the monthly traffic volume data of a certain road over the years, and establish the monthly traffic volume time series of the road
[0064] In this embodiment, taking the monthly traffic volume time series of a road in Guangxi Province as an example, the traffic volume data from January 2014 to December 2018 are used as historical value fitting samples, and the traffic volume data from January 2019 to December 2019 The data is used as a comparison sample for prediction.
[0065] 2) Convert the monthly traffic volume time series Use the SARIMA model for linear prediction and get linear results
[0066] Since the traffic volume series is monthly data and has obvious seasonal trends, the number of periods is taken as m =12. At the same time, considering that it is a non-stationary time series, differential processing is required ...
PUM
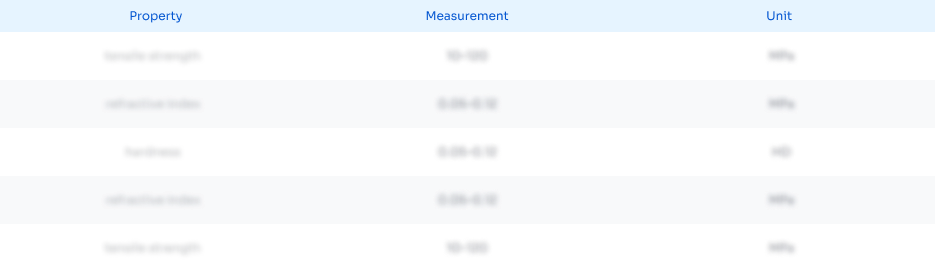
Abstract
Description
Claims
Application Information
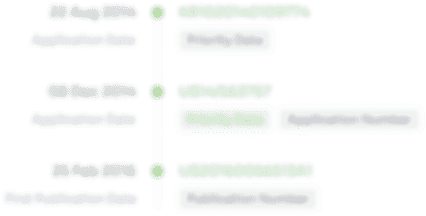
- R&D Engineer
- R&D Manager
- IP Professional
- Industry Leading Data Capabilities
- Powerful AI technology
- Patent DNA Extraction
Browse by: Latest US Patents, China's latest patents, Technical Efficacy Thesaurus, Application Domain, Technology Topic, Popular Technical Reports.
© 2024 PatSnap. All rights reserved.Legal|Privacy policy|Modern Slavery Act Transparency Statement|Sitemap|About US| Contact US: help@patsnap.com