Deep learning defect image recognition method and system based on ensemble learning
A deep learning and image recognition technology, applied in the field of deep learning defect image recognition methods and systems, can solve the problems of poor generality of recognition models, detection failures, missed detections, etc., and achieve the effect of improving recognition accuracy and high versatility
- Summary
- Abstract
- Description
- Claims
- Application Information
AI Technical Summary
Problems solved by technology
Method used
Image
Examples
Embodiment Construction
[0087] In some processes described in the specification and claims of the present invention and the above-mentioned drawings, a plurality of operations appearing in a specific order are contained, but it should be clearly understood that these operations may not be performed in the order in which they appear herein Execution or parallel execution, the serial numbers of the operations, such as 101, 102, etc., are only used to distinguish different operations, and the serial numbers themselves do not represent any execution order. Additionally, these processes can include more or fewer operations, and these operations can be performed sequentially or in parallel. It should be noted that the descriptions of "first" and "second" in this article are used to distinguish different messages, devices, modules, etc. are different types.
[0088] The following will clearly and completely describe the technical solutions in the embodiments of the present invention with reference to the a...
PUM
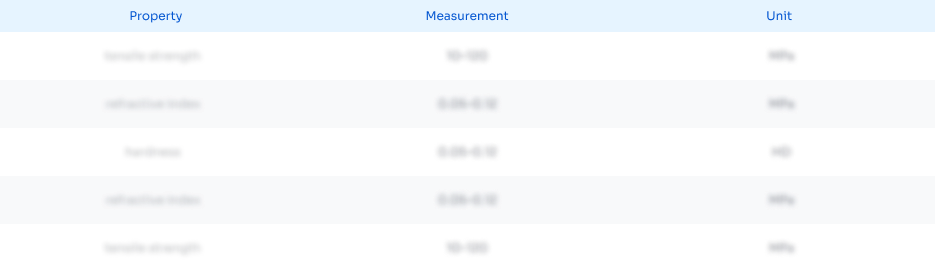
Abstract
Description
Claims
Application Information
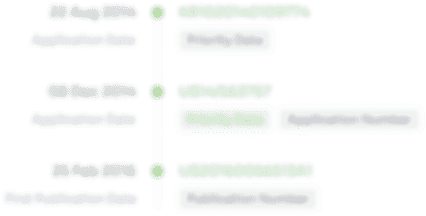
- R&D Engineer
- R&D Manager
- IP Professional
- Industry Leading Data Capabilities
- Powerful AI technology
- Patent DNA Extraction
Browse by: Latest US Patents, China's latest patents, Technical Efficacy Thesaurus, Application Domain, Technology Topic.
© 2024 PatSnap. All rights reserved.Legal|Privacy policy|Modern Slavery Act Transparency Statement|Sitemap