Power grid geological settlement hidden danger risk prediction method based on machine learning
A technology of machine learning and risk prediction, which is applied in the directions of prediction, instrumentation, and data processing applications, etc., and can solve problems such as failure to discover hidden dangers of geological subsidence in the power grid, untimely investigation of hidden dangers, economic losses, and reduced efficiency of power grid operation and maintenance personnel.
- Summary
- Abstract
- Description
- Claims
- Application Information
AI Technical Summary
Problems solved by technology
Method used
Image
Examples
Embodiment
[0035] see figure 1 , is a flow chart of a machine learning-based risk prediction method for geological subsidence hazards in power grids.
[0036] like figure 1 As shown, the present application provides a machine learning-based method for predicting the risk of hidden dangers of geological subsidence in power grids, which specifically includes the following steps:
[0037] S101. Determine the geographic area of the grid, and divide the geographic area of the grid into geographic grids at preset intervals, wherein the geographic grids include sample geographic grids and to-be-predicted geographic grids, and the sample geographic grids Include the first feature data and the first predictor variable corresponding to the first feature data, the first predictor variable is a known variable, the geographic grid to be predicted includes the second feature data and the A second predictor variable corresponding to the second feature data, where the second predictor variable is ...
PUM
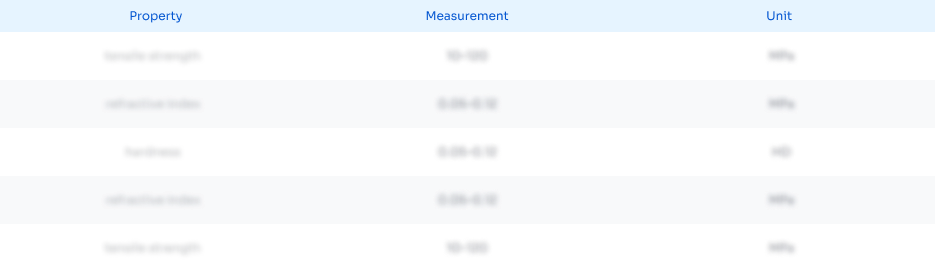
Abstract
Description
Claims
Application Information
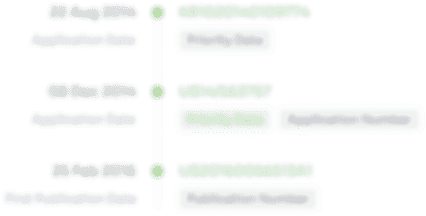
- R&D Engineer
- R&D Manager
- IP Professional
- Industry Leading Data Capabilities
- Powerful AI technology
- Patent DNA Extraction
Browse by: Latest US Patents, China's latest patents, Technical Efficacy Thesaurus, Application Domain, Technology Topic, Popular Technical Reports.
© 2024 PatSnap. All rights reserved.Legal|Privacy policy|Modern Slavery Act Transparency Statement|Sitemap|About US| Contact US: help@patsnap.com