Few-sample image classification method and system based on mutual neighbors
A technology of sample images and classification methods, which is applied in the directions of instruments, calculations, character and pattern recognition, etc., can solve problems such as costing a lot of manpower and not having enough training samples, and achieve the effect of eliminating interference and improving accuracy
- Summary
- Abstract
- Description
- Claims
- Application Information
AI Technical Summary
Problems solved by technology
Method used
Image
Examples
Embodiment Construction
[0058] The present invention will be further described in detail below with reference to the accompanying drawings and embodiments. It should be noted that the following embodiments are intended to facilitate the understanding of the present invention, but do not limit it in any way.
[0059] Such as figure 1 As shown, the main model of the present invention is divided into a visual feature module, a mutual neighbor screening module, and a similarity calculation module, and the final similarity calculation score is used for the optimization process of the entire module. Specific steps are as follows:
[0060] Step 1. The visual feature module learns the deep visual feature θ of the input image x during the few-shot image classification training process. The basic steps are as follows:
[0061] 1-1. The query image in the i-th task in the few-shot image classification task is first cropped and scaled to an image x of size 84×84 (i) As the query input of the network; the suppo...
PUM
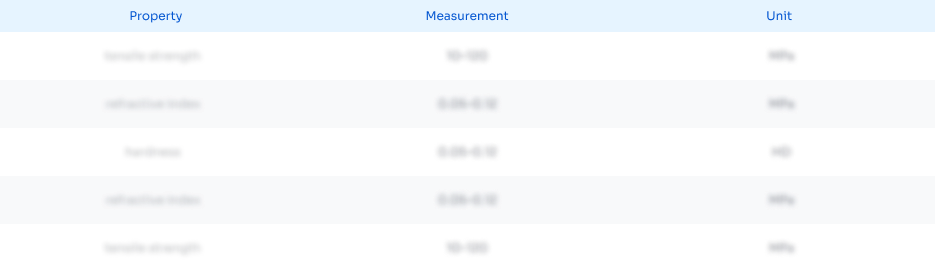
Abstract
Description
Claims
Application Information
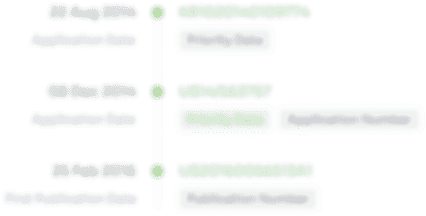
- R&D Engineer
- R&D Manager
- IP Professional
- Industry Leading Data Capabilities
- Powerful AI technology
- Patent DNA Extraction
Browse by: Latest US Patents, China's latest patents, Technical Efficacy Thesaurus, Application Domain, Technology Topic, Popular Technical Reports.
© 2024 PatSnap. All rights reserved.Legal|Privacy policy|Modern Slavery Act Transparency Statement|Sitemap|About US| Contact US: help@patsnap.com